AI-enabled Testing Market – Global Industry Size, Share, Trends, Opportunity, and Forecast, Segmented By Component (Solution, Services), By Deployment (Cloud, On-premise), By End-use Industry (Government, BFSI, IT & Telecommunication, Energy & Utility, Others), By Application (Test Automation, Infrastructure Optimization, Others), By Technology (Machine Learning and Pattern Recognition, Natural La
Published Date: November - 2024 | Publisher: MIR | No of Pages: 320 | Industry: ICT | Format: Report available in PDF / Excel Format
View Details Buy Now 2890 Download Sample Ask for Discount Request CustomizationForecast Period | 2024-2028 |
Market Size (2022) | USD 425 Million |
CAGR (2023-2028) | 17% |
Fastest Growing Segment | Test Automation |
Largest Market | North America |
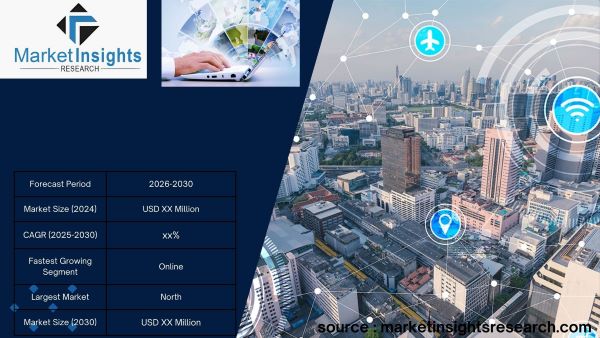
Market Overview
Global AI-enabled Testing Market has valued at USD 425 Million in 2022 and is anticipated to project robust growth in the forecast period with a CAGR of 17% through 2028. The global AI-enabled testing market is currently experiencing robust growth, underpinned by a convergence of factors reshaping the software testing landscape. As software applications become increasingly complex and intricate, traditional testing methods struggle to keep pace. This challenge has paved the way for AI-enabled testing solutions to emerge as a critical component of the software development lifecycle. AI leverages machine learning algorithms and automation to enhance testing processes, resulting in greater efficiency, accuracy, and speed. These solutions excel at identifying defects, predicting potential issues, and optimizing test coverage, all while reducing manual intervention. This transformative shift has proven particularly beneficial for businesses striving to meet the demands of agile development and continuous integration/continuous deployment (CI/CD) pipelines, ensuring that software is not only delivered faster but with higher quality and reliability.
Furthermore, the rising adoption of AI-enabled testing can be attributed to the growing diversity of platforms, devices, and operating systems in the digital landscape. The need to ensure seamless functionality across a multitude of configurations necessitates more comprehensive and adaptive testing methodologies. AI's ability to simulate real-world scenarios, automate test case generation, and analyze vast datasets empowers organizations to achieve this level of thoroughness. Additionally, AI-driven testing contributes to cost savings by reducing testing cycles, minimizing the risk of software failures, and improving overall product quality. As businesses across industries recognize the advantages of AI-enabled testing in delivering superior customer experiences and maintaining competitive edge, the global market for these solutions is poised for continued expansion and innovation.
Key Market Drivers
Enhanced Testing Efficiency
AI-enabled testing is transforming the software development lifecycle by automating repetitive and time-consuming testing tasks, allowing testers to focus on more complex and critical areas. With the help of AI algorithms, large volumes of test data can be analyzed, patterns can be identified, and test cases can be generated, reducing the manual effort required for test design and execution. This significantly improves testing efficiency and accelerates the overall software development lifecycle. One of the key benefits of AI-enabled testing is its ability to automate test case generation. AI algorithms can analyze historical test data, identify patterns, and generate test cases that cover a wide range of scenarios. This eliminates the need for manual test case creation, saving time and effort for testers. Moreover, AI algorithms can continuously learn from test results and refine the test cases over time, ensuring that the testing process remains up-to-date and effective.
In addition to test case generation, AI-enabled testing also enhances test execution. AI algorithms can analyze test results in real-time, identify patterns of failures, and prioritize the most critical issues. This allows testers to focus their attention on resolving high-priority issues, improving the overall quality of the software. Furthermore, AI algorithms can detect anomalies and deviations from expected behavior, enabling early detection of potential defects and reducing the risk of releasing faulty software. AI-enabled testing also plays a crucial role in test analysis and reporting. AI algorithms can analyze test results, identify trends, and provide insights into the quality of the software. This helps stakeholders make informed decisions about the readiness of the software for release. Additionally, AI algorithms can generate comprehensive test reports, highlighting the areas that require further attention and providing recommendations for improvement.
However, it is important to note that AI-enabled testing is not meant to replace human testers. Human expertise is still essential in test design, analysis, and decision-making. AI-enabled testing should be seen as a tool that augments the capabilities of testers, allowing them to focus on more complex and critical aspects of testing.
Improved Test Coverage
AI-enabled testing harnesses the power of AI algorithms to analyze large volumes of data and identify potential risks and vulnerabilities in software applications. By leveraging AI algorithms, organizations can achieve broader test coverage, ensuring that all critical functionalities and scenarios are thoroughly tested. This comprehensive approach reduces the likelihood of software defects and enhances the overall quality of the product. AI algorithms can analyze complex code structures, identify potential bugs, and even predict areas of the software that are prone to failure. This enables organizations to proactively address these issues before they impact end-users. Additionally, AI-enabled testing can simulate real-world scenarios and user interactions, allowing organizations to validate the performance and reliability of their software under different conditions. By uncovering potential issues early in the development process, organizations can save time and resources by addressing them before they become more challenging and costly to fix. Furthermore, AI algorithms can continuously learn from test results and adapt their testing strategies, improving the effectiveness and efficiency of future testing cycles. This iterative learning process enables organizations to refine their testing approaches and optimize their software quality assurance efforts. Overall, AI-enabled testing empowers organizations to achieve higher levels of software quality, reduce the risk of defects, and deliver robust and reliable products to their customers.
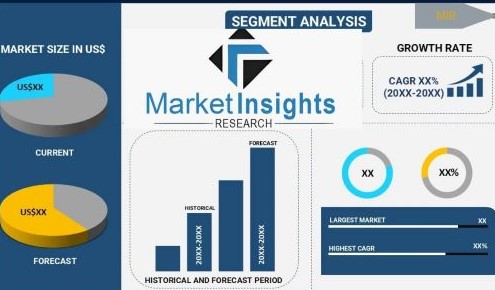
Intelligent Test Case Generation
AI-enabled testing leverages machine learning algorithms to generate test cases based on historical data, user behavior, and system usage patterns. By analyzing past test results, these algorithms can identify areas that require additional testing, allowing testers to focus their efforts on high-risk areas. This intelligent test case generation enhances test coverage and helps identify defects early in the development process.
The use of AI algorithms in test case generation brings several benefits to the software development lifecycle. Firstly, it reduces the manual effort required for test case creation. Traditionally, testers had to manually design and create test cases, which could be time-consuming and prone to human error. With AI-enabled testing, machine learning algorithms can analyze historical data and automatically generate test cases that cover a wide range of scenarios. This not only saves time but also ensures that test cases are comprehensive and cover critical functionalities. Secondly, AI-enabled test case generation improves test coverage. By analyzing past test results, machine learning algorithms can identify areas of the software that have not been adequately tested or have a higher likelihood of containing defects. Testers can then focus their efforts on these high-risk areas, ensuring that potential issues are identified and addressed early in the development process. This targeted approach to test case generation enhances the overall quality of the software and reduces the risk of releasing faulty products.
Furthermore, AI algorithms can continuously learn and adapt based on test results. As new test data becomes found at, the algorithms can analyze it and refine the test cases generated. This iterative learning process allows the algorithms to improve their accuracy and effectiveness over time, resulting in more efficient and effective test case generation.
Predictive Analytics for Defect Prevention
AI algorithms have the capability to analyze historical data and detect patterns that are indicative of software defects. By harnessing the power of predictive analytics, organizations can proactively identify potential defects and implement preventive measures to mitigate risks. This proactive approach plays a crucial role in reducing the number of defects and enhancing the overall quality of the software. The ability of AI algorithms to analyze historical data is instrumental in identifying patterns that are associated with software defects. By examining past test results, bug reports, and other relevant data, these algorithms can uncover correlations and trends that indicate the presence of defects. This deep analysis enables organizations to gain valuable insights into the root causes of defects and the factors that contribute to their occurrence. By leveraging predictive analytics, organizations can go beyond simply identifying defects and take proactive measures to prevent their occurrence. AI algorithms can use the patterns identified from historical data to predict the likelihood of future defects. This predictive capability empowers organizations to anticipate potential issues and implement preventive measures before they manifest as actual defects. By addressing the underlying causes and mitigating risks in advance, organizations can significantly reduce the number of defects that occur during the software development process.
The implementation of preventive measures based on predictive analytics helps organizations improve the overall software quality. By identifying and addressing potential defects early on, organizations can avoid costly rework, delays, and customer dissatisfaction. Moreover, the proactive approach enables organizations to optimize their resources and allocate them more effectively towards defect prevention rather than reactive defect resolution. In addition to reducing the number of defects, leveraging predictive analytics also enhances the efficiency of the software development process. By proactively identifying potential defects, organizations can streamline their testing efforts, prioritize critical areas, and allocate resources more efficiently. This leads to faster development cycles, improved time-to-market, and increased customer satisfaction.
Test Automation and Continuous Testing
AI-enabled testing plays a crucial role in automating various aspects of the testing process, including test case generation, execution, and analysis. This automation empowers organizations to implement continuous testing practices, where tests are executed continuously throughout the software development lifecycle. Continuous testing brings several benefits, including faster feedback, early defect detection, and faster time-to-market.
One of the key advantages of AI-enabled testing is the automation of test case generation. AI algorithms can analyze historical data, user behavior, and system usage patterns to automatically generate test cases. This eliminates the need for manual test case creation, saving time and effort for testers. Moreover, AI algorithms can continuously learn from test results and refine the test cases over time, ensuring that the testing process remains up-to-date and effective. In addition to test case generation, AI-enabled testing facilitates the automation of test execution. With the help of AI algorithms, tests can be executed automatically, without the need for manual intervention. This automation enables organizations to execute tests more frequently and consistently, ensuring that the software is thoroughly tested throughout the development process. By continuously running tests, organizations can receive faster feedback on the quality of the software, allowing them to identify and address defects early on.
Furthermore, AI-enabled testing enables organizations to automate the analysis of test results. AI algorithms can analyze test data, identify patterns, and provide insights into the quality of the software. This automated analysis helps organizations quickly identify potential issues and make informed decisions about the readiness of the software for release. By detecting defects early in the development process, organizations can reduce the time and effort required for defect resolution, leading to faster time-to-market. By implementing continuous testing practices through AI-enabled testing, organizations can achieve faster feedback, early defect detection, and faster time-to-market. Continuous testing ensures that tests are executed continuously throughout the software development lifecycle, enabling organizations to identify and address issues early on. This iterative and automated approach to testing improves the overall quality of the software, reduces the risk of releasing faulty products, and accelerates the delivery of software to the market.
Key Market Challenges
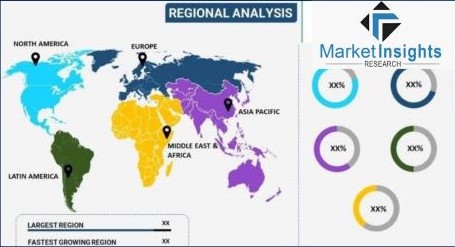
Lack of Awareness and Understanding
A significant challenge facing the global AI-enabled testing market is the limited awareness and understanding among organizations regarding the importance and advantages of adopting AI-driven testing solutions. Many businesses, especially smaller enterprises, may not fully grasp the potential risks and vulnerabilities associated with traditional testing methods and the potential for AI to enhance testing efficiency and accuracy. This lack of awareness can lead to hesitation in investing in AI-enabled testing, leaving organizations vulnerable to testing deficiencies and software errors. Addressing this challenge requires comprehensive educational initiatives to highlight the critical role that AI plays in test automation, defect detection, and overall software quality improvement. Organizations need to recognize that inadequate testing can result in costly errors, product delays, and damage to their reputation. Real-world examples and case studies showcasing the tangible benefits of AI-enabled testing can help foster a deeper understanding of its significance.
Complexity and Integration Issues
The implementation and management of AI-enabled testing solutions can pose complex challenges for organizations, especially those with limited IT resources or expertise. Configuring AI testing systems effectively and integrating them with existing testing processes and tools can be technically demanding. Compatibility issues may arise during integration, leading to delays and suboptimal performance. To address these challenges, it's essential to simplify the deployment and management of AI-enabled testing solutions. User-friendly interfaces and intuitive configuration options should be provided to streamline setup and customization. Additionally, organizations should have access to comprehensive support and guidance, including documentation, tutorials, and technical experts who can assist with integration and troubleshoot any issues. Simplifying these aspects of AI-enabled testing implementation can lead to more efficient processes and improved testing outcomes.
Overcoming False Positives and Performance Impact
AI-enabled testing systems are crucial for identifying potential defects and issues, but they face the challenge of false positives—identifying legitimate aspects as problematic. These false positives can disrupt testing workflows and lead to frustration among testing teams. Additionally, the performance of AI-enabled testing solutions can impact the overall testing process, especially when handling large volumes of test cases. Striking the right balance between rigorous testing and minimizing false positives while ensuring optimal performance is essential. To overcome this challenge, ongoing refinement of AI algorithms and technologies is necessary. Improved algorithms can reduce false positives by accurately distinguishing between genuine defects and non-issues. Optimization of AI-enabled testing systems can minimize latency and ensure efficient testing, even under heavy workloads. Continuous improvement in these areas will enable organizations to benefit from AI-enabled testing while maintaining a smooth and reliable testing process, enhancing software quality.
Key Market Trends
Rise in Sophisticated Cyber Attacks
The global market for AI-enabled Testings (WAFs) is experiencing an increase in the number of sophisticated cyber attacks specifically targeting web applications. Hackers are continuously evolving their techniques to exploit vulnerabilities and gain unauthorized access to sensitive data. Consequently, there is a rising demand for advanced WAF solutions that can effectively detect and mitigate these sophisticated attacks. In response to this demand, WAF vendors are concentrating their efforts on developing intelligent and adaptive solutions that possess the capability to analyze traffic patterns, identify anomalies, and offer real-time protection against emerging threats. These advanced WAF solutions leverage technologies such as artificial intelligence and machine learning to enhance their threat detection capabilities. By analyzing vast amounts of data, these solutions can identify patterns and detect anomalies in real-time, enabling organizations to respond swiftly to evolving threats. The goal is to provide organizations with proactive defense mechanisms that can adapt to the ever-changing threat landscape. With the continuous advancements in WAF technologies, organizations can benefit from robust security measures that effectively safeguard their web applications and protect sensitive data from unauthorized access. By investing in intelligent and adaptive WAF solutions, organizations can stay one step ahead of cyber attackers and ensure the integrity and confidentiality of their web applications.
Shift towards Cloud-based WAF Solutions
The global market is experiencing a significant shift towards cloud-based AI-enabled Testing (WAF) solutions. This shift is driven by the increasing adoption of cloud computing and the migration of applications to the cloud. Organizations are now seeking WAF solutions that can seamlessly integrate with their cloud infrastructure to ensure comprehensive security for their web applications.
Cloud-based WAF solutions offer several advantages. Firstly, they provide scalability, allowing organizations to easily adjust their resources based on the changing demands of their web applications. This scalability ensures that the WAF solution can handle varying levels of traffic and effectively protect the applications during peak usage periods.
Cloud-based WAF solutions offer flexibility. They can be easily deployed and managed across multiple cloud environments, providing organizations with the freedom to choose the cloud platform that best suits their needs. This flexibility enables seamless integration with existing cloud infrastructure and ensures that the WAF solution can adapt to the specific requirements of the organization.
Integration of Artificial Intelligence and Machine Learning
The integration of artificial intelligence (AI) and machine learning (ML) technologies into WAF solutions is a significant trend in the market. AI and ML algorithms can analyze vast amounts of data, identify patterns, and detect anomalies in real-time, enabling WAF solutions to adapt and respond to evolving threats effectively. These advanced technologies enhance the accuracy and efficiency of WAF solutions, reducing false positives and false negatives. WAF vendors are investing in AI and ML capabilities to enhance threat detection, automate security operations, and provide proactive defense against emerging threats.
Segmental Insights
Component Insights
The software segment held the highest revenue share of over 77% in 2022. SMEsfrequently employ AI testing services to evaluate the effectiveness of software programs. For SMEs, AI testing services offer many advantages, including lower costs, more efficiency, and improved user experience. Moreover, organizations streamline preparing, managing, and analyzing test data by leveraging data-wrangling software in AI-enabled testing. This integration enhances testing accuracy, efficiency, and effectiveness by ensuring that the correct data is found at in a suitable format, enabling comprehensive test coverage and reliable results.The service segment is estimated to grow at the highest CAGR over the forecast period.
The significant advancement of managed and professional services accounts for this growth. AI-enabled testing tools often requires significant computational resources and infrastructure to support complex algorithms and large-scale testing. Managed services providers like QA Mentor, Testlio, Capgemini, and others offer the necessary infrastructure, such as cloud-based platforms or dedicated environments, to support AI testing initiatives. They also scale resources based on demand, ensuring optimal performance, quality monitoring, and cost-efficiency; for instance, Capgemini’s embedded quality engineering in “ADMnext,” a whole stack of Application Development and Maintenance (ADM) services, have the capabilities and solutions to address a wide array of challenges and help the user make better business decisions ensuring better performance.
Deployment
The on-premises segment led the market in 2022 accounting for over 61% of the global revenue. On-premises environments require the necessary infrastructure to support AI-enabled testing. This comprises setting up servers, storage, and networking capabilities to handle the computational requirements of AI algorithms and models. Organizations collect, store, and manage the relevant data required for AI-enabled testing within their on-premises infrastructure. Data collection involves ensuring data security, privacy, and compliance with regulations governing the handling of sensitive or confidential information. Moreover, organizations must customize or develop connectors, plugins, or interfaces to integrate AI capabilities into their existing testing tools, frameworks, or processes to ensure smooth collaboration and integration of AI techniques with the on-premises testing environment fueling the market growth.
The cloud segment is estimated to grow at the highest CAGR over the forecast period. This growth is led by cloud-based solutions offering virtually unlimited scalability and resources. Organizations efficiently deliver and scale up or down their AI-enabled testing infrastructure based on demand. This flexibility allows them to handle large-scale testing requirements efficiently and cost-effectively. Moreover, cloud-based AI-enabled testing tools can seamlessly integrate with other cloud services, such as cloud-based test management tools, version control systems, bug tracking systems, and continuous integration/continuous deployment (CI/CD) pipelines. This integration streamlines the software development and testing process, enhancing efficiency and collaboration.
Application Insights
The test automation segment held the largest revenue share of over 58% in 2022. The growth is led by AI-testing tools, which greatly enhance test automation by leveraging Artificial Intelligence(AI) algorithms and techniques. Combined with cloud computing instances, it can bring even more scalability and flexibility to the testing process. By combining AI-enabled testing with test automation, organizations achieve higher efficiency, accuracy, and productivity in their testing processes. AI algorithms also enhance test case generation, data management, test execution, analysis, and predictive capabilities, augmenting the capabilities of test automation tools and frameworks.
This ultimately leads to improved software quality, faster time-to-market, and better overall testing outcomes fueling the market growth.The infrastructure optimization segment is anticipated to showcase significant growth over the forecast period. Organizations leveraging AI techniques improve their testing infrastructure's efficiency, scalability, and cost-effectiveness. Rapid advancement in cloud infrastructure has enabled the IT Infrastructure to be flexible, intangible, and on-demand. AI algorithms automatically generate test cases based on the system's analysis under test, reduce the manual effort required for test case creation, and ensure comprehensive test coverage. Moreover, AI prioritizes test cases based on code changes, defect history, or criticality, allowing organizations to focus their testing efforts on high-priority areas
Regional Insights
North America dominated the market in 2022, accounting for over 39% share of the global revenue. Prominent growth of automation testing is fueling the market growth in this region. AI regression testing is increasingly being used in mobile applications, influencing AI-enabled testing in North America as they improve the product's functionality. Moreover, the United States is expected to advance significantly over the forecast period due to technology providers' presence. Increasing urbanization, changing lifestyles, rising disposable income, and advanced technologies fuel the market growth in this region.
Increasing investment in R&D activities, increasing preference for automated testing solutions, and launching new products are also driving the market growth in the U.S. Asia Pacific is anticipated to witness significant CAGR growth over the forecast period. India, China, Japan, and other countries of Asia Pacific are innovating and launching new products and platforms to fuel market growth. Major innovations of 5G in Japan are propelling market growth in this region. The usage of AI-enabled testing technologies in Japan may increase because of a potential spike in demand for automated and efficient telecom infrastructure testing and maintenance. Moreover, In June 2022, Singapore launched the first AI governance testing framework and toolbox to assist AI developers in objectively and independently evaluating their systems.
Recent Developments
- InJuly 2023, PQR Testing Solutions Unveiled TestMaster AI 2.0. PQR TestingSolutions, a prominent player in the AI-enabled testing market, introduced thelatest iteration of their AI-driven testing platform, TestMaster AI 2.0. Thisupdated solution incorporates enhanced machine learning algorithms andpredictive analytics to further streamline the testing process. TestMaster AI2.0 offers improved test case generation, increased test coverage, and fasterdefect identification. It also boasts new integrations with popular CI/CDtools, making it easier for organizations to integrate AI-enabled testingseamlessly into their development pipelines.
- InJune 2023, LMN Test Labs Released AI TestBench LMN Test Labs, a leading providerof AI-enabled testing solutions, launched AI TestBench, a comprehensive testingenvironment designed to facilitate the development and execution of AI-driventest scripts. AI TestBench includes a user-friendly interface for creating andmanaging test scenarios, as well as pre-built AI models for common testingtasks. This development aims to empower testing teams to leverage AI moreeffectively in their testing efforts, accelerating test automation andimproving overall software quality.
- InMarch 2023, RST Technologies Introduced AI TestOps RST Technologies unveiled AITestOps, a novel AI-enabled testing platform focused on optimizing testorchestration and management. AI TestOps leverages machine learning todynamically allocate testing resources, prioritize test execution, and identifytesting bottlenecks in real time. This innovation aims to enhance testingefficiency, reduce testing costs, and ensure timely delivery of high-qualitysoftware, aligning with the growing emphasis on DevOps and continuous testingpractices.
- InNovember 2022, UVW Software Solutions Launched TestAI Insights UVW SoftwareSolutions expanded their AI-enabled testing portfolio with the introduction ofTestAI Insights. This solution incorporates advanced data analytics andvisualization capabilities to provide testing teams with actionable insightsderived from testing data. TestAI Insights enables organizations to identifytesting trends, optimize testing strategies, and make informed decisions basedon comprehensive testing performance metrics. This development reflects theincreasing importance of data-driven decision-making in software testing.
Key Market Players
- Sauce Labs Inc.
- ReTest GmbH
- D2L Corp.
- Functionize Inc.
- Diffblue Ltd.
- Applitools
- Capgemini SE
- testRigor
- Micro Focus International Plc
- Tricentis
By Component | By Deployment | By End-use Industry | By Application | By Technology | By Region |
|
|
|
|
|
|
Related Reports
- SVOD Market Size - By Content (General Entertainment, Niche Content, Original Programming), By Pricing Model (Premium, A...
- Multimodal AI Market Size - By Component (Solution, Service), By Technology (Machine Learning, Natural Language Processi...
- AVOD Market Size - By Content (Movies, TV Shows, Original Series, News, Sports, User-generated Content), By Platform (S...
- Premium Finance Market - By Type (Life Insurance, Non-life Insurance), By Interest Rate (Fixed Interest Rate, Floating I...
- Gift Cards Market - By Type (Closed-loop Gift Cards, Open-loop Gift Cards), By Distribution Channel (Online, Offline), B...
- Biometric Payment Market Size - By Biometric Mode (Fingerprint Recognition, Facial Recognition, Voice Recognition, Iris ...
Table of Content
To get a detailed Table of content/ Table of Figures/ Methodology Please contact our sales person at ( chris@marketinsightsresearch.com )
List Tables Figures
To get a detailed Table of content/ Table of Figures/ Methodology Please contact our sales person at ( chris@marketinsightsresearch.com )
FAQ'S
For a single, multi and corporate client license, the report will be available in PDF format. Sample report would be given you in excel format. For more questions please contact:
Within 24 to 48 hrs.
You can contact Sales team (sales@marketinsightsresearch.com) and they will direct you on email
You can order a report by selecting payment methods, which is bank wire or online payment through any Debit/Credit card, Razor pay or PayPal.
Discounts are available.
Hard Copy