Artificial Intelligence Chipset Market – Global Industry Size, Share, Trends, Opportunity, and Forecast, Segmented by Type of AI Chipset (GPU (Graphics Processing Unit) AI Chipsets, CPU (Central Processing Unit) AI Chipsets), FPGA (Field-Programmable Gate Array) AI Chipsets, ASIC (Application-Specific Integrated Circuit) AI Chipsets) By Technology (Deep Learning, Machine Learning, Quantum AI Chips
Published Date: November - 2024 | Publisher: MIR | No of Pages: 320 | Industry: ICT | Format: Report available in PDF / Excel Format
View Details Buy Now 2890 Download Sample Ask for Discount Request CustomizationForecast Period | 2024-2028 |
Market Size (2022) | USD 18.45 billion |
CAGR (2023-2028) | 28.76% |
Fastest Growing Segment | Machine Learning |
Largest Market | North America |
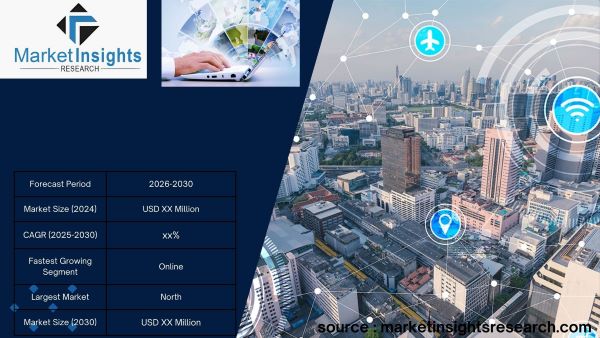
Market Overview
Global Artificial Intelligence Chipset Market has experienced tremendous growth in recent years and is poised to continue its strong expansion. The Artificial Intelligence Chipset Market reached a value of USD 18.45 billion in 2022 and is projected to maintain a compound annual growth rate of 28.76% through 2028.The artificial intelligence chipset market has seen tremendous growth in recent years as organizations increasingly leverage AI and wearable technologies to gain insights and automate processes. Powered by advancements in AI chipset capabilities, businesses are finding new ways to optimize operations, engage talent, and enhance customer experiences.One major area of adoption is in talent management. Platforms utilizing AI chipsets in wearable devices can now provide unprecedented visibility into workforce performance. Tools like augmented and virtual reality headsets equipped with AI chipsets allow companies to monitor behaviors and detect anomalies in real-time. These behavioral analytics powered by AI chipsets helps address challenges such as fraud prevention and regulatory compliance. Financial institutions have been early adopters of these technologies. As remote and hybrid work models become prevalent, data-driven oversight of global operations is more crucial. Leading companies are leveraging mixed reality data and AI-powered wearables to streamline collaboration between distributed teams. This enables more effective engagement of remote employees and digital-first customers. AI chipset providers continue investing heavily in predictive modeling, AI integration, and user-friendly designs. This will allow even greater value from wearables going forward. Applications such as predictive maintenance, optimized decision-making, and personalized digital services for customers are well-positioned to grow. The talent management and customer experience markets remain strong growth opportunities for AI chipset vendors as wearables integrate more advanced AI capabilities. This fuels personalized insights and automated processes addressing evolving workforce and customer needs in an increasingly digital world. The artificial intelligence chipset market outlook remains positive.
Key Market Drivers
Growing Demand for AI Processing Power
One of the primary drivers fueling growth in the AI chipset market is the rising computational requirements for advanced AI workloads. As AI algorithms become more sophisticated, they generate exponentially greater amounts of data and require faster processing speeds. Traditional CPU and GPU chips struggle to keep pace with these intensifying demands. AI chipsets like ASICs, FPGAs and neuromorphic chips have emerged as a solution, designed specifically for high-performance AI tasks. They offer capabilities like parallel processing, in-memory computing and low-power operation ideal for AI. As companies across industries increasingly adopt AI to gain competitive advantages, there will be escalating needs for specialized AI silicon with exponentially higher throughput, efficiency and lower latency than mainstream chips. This growing computational demand is opening many opportunities for AI chipset vendors.
Integration of AI into IoT Devices and Edge Computing
The integration of AI capabilities into internet-connected devices and edge systems is another key driver. As more 'things' become intelligent with embedded AI, there will be vast requirements for low-power, compact AI chipsets that can operate at the IoT edge with limited resources. Applications like predictive maintenance, computer vision, speech recognition and autonomous vehicles will drive this trend. AI chipsets designed for embedded and edge deployments allow advanced analytics to occur locally on devices rather than sending all data to the cloud. This delivers benefits like reduced latency, lower bandwidth usage, enhanced privacy and operational cost savings. It also enables entirely new AI use cases requiring real-time, on-device processing. The proliferation of AI into billions of IoT endpoints and edge nodes worldwide will be a significant growth accelerator for specialized AI chipset vendors.
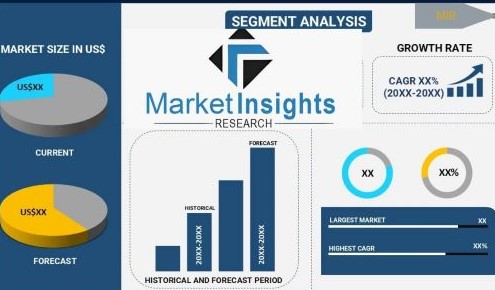
Increasing Government Initiatives for National AI Strategies
Key Market Challenges
Design Complexity Hurdles
One of the key challenges AI chipset vendors face is the immense complexity involved in designing specialized silicon for advanced AI workloads. Unlike general-purpose chips, AI chips require novel architectures and capabilities optimized for tasks like neural network training and inference. This involves developing application-specific instruction sets, memory hierarchies, interconnects and processing elements from the ground up. Achieving high throughput and efficiency while maintaining flexibility to support evolving AI algorithms also poses major technical difficulties. Ensuring chips can be programmed and integrated easily presents further complications. Any defects or design flaws could significantly impact performance and user experience. The multi-year chip design process also makes it hard to quickly adapt to shifting market needs. While specialized AI chips deliver performance advantages, low-level design challenges will continue hampering innovation efforts and time-to-market speeds, restricting revenue opportunities in the near term. Overcoming these obstacles through ongoing R&D investments and design expertise will be critical for chipset vendors.
Talent Shortages Hamper Growth
A significant constraint on the AI chipset industry is the global shortage of specialized semiconductor talent, including chip architects, hardware engineers and AI algorithm experts. Developing cutting-edge AI silicon requires deep skills that are still emerging areas of study. While demand is surging from companies seeking AI solutions, the supply of qualified talent has failed to keep pace. This talent crunch limits the scale and speed of new product development cycles. It also hinders the pursuit of advanced process technologies like 3nm and below that are crucial for future AI chips. The talent shortage is exacerbated by intense competition among technology giants for semiconductor professionals. Although training programs and university partnerships can help address this, attracting and retaining top talent remains a pressing concern for the long-term success of the AI chipset sector. Strategic collaborations and competitive compensation practices will be needed to overcome this human capital challenge.
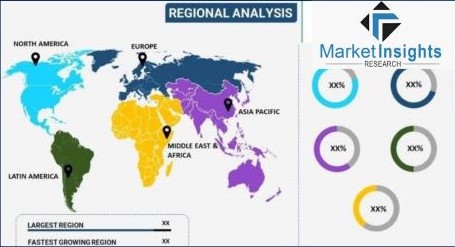
Key Market Trends
Rise of Neuromorphic Computing Chips
Neuromorphic computing, which aims to emulate neural architectures of the human brain, is gaining significant traction as a promising new paradigm for advanced AI workloads. Traditional von Neumann architecture chips face limitations in power efficiency and scalability for tasks like complex pattern recognition. Neuromorphic chips composed of neurons and synapses can perform these functions using just a fraction of the energy. Startups like Intel's Loihi, IBM's TrueNorth, and BrainChip are developing neuromorphic AI accelerators focused on low-power edge/IoT applications. Meanwhile, research initiatives like the EU Human Brain Project are making progress in large-scale neuromorphic systems. This trend poses a disruptive threat to incumbent AI chip vendors reliant on conventional architectures. While technical challenges remain, successful commercialization of neuromorphic AI chips could open new markets around embedded vision, speech recognition, and other power-constrained AIoT uses over the next 5 years. It may also lead to specialized neuromorphic processors for datacenter training in the longer term.
Proliferation of Edge-Optimized AI Accelerators
With AI becoming ubiquitous in IoT devices and edge systems, there is growing demand for dedicated AI accelerators designed specifically for on-device and local processing. These edge AI chips optimize performance per watt and minimize latency for real-time AI tasks like computer vision, speech recognition, and natural language processing. They feature low-power processor cores combined with specialized hardware accelerators for common AI operations. Companies like Nvidia, Intel, and Xilinx are investing heavily in edge-focused AI SoCs and modules. Startups like Mythic, Anthropic, and Flex Logix have also emerged targeting this space. Mass production of such accelerators will be critical to power intelligent systems across industries like smart cities, healthcare, retail, and industrial automation over the next decade. This trend is expected to drive over 50% of AI chip revenues by 2030 as cloud-style AI moves closer to billions of distributed edge devices.
Emergence of AI Chip Multicore Architectures
As AI models grow exponentially in size and complexity, the demand for parallel processing power is intensifying. Traditional single/dual-core AI chips are reaching their computational limits. This is driving the rise of multicore AI chip architectures with tens to hundreds of specialized cores optimized for distributed deep learning. Startups like Graphcore and Cerebras Systems have pioneered this approach in datacenter AI training chips featuring over 1000 cores. Meanwhile, companies like Intel, AMD, and Nvidia are integrating dozens of AI cores into mainstream CPUs and GPUs. Massively multicore AI chips promise 10-100x gains in AI performance at lower costs versus single/few core designs. They are poised to accelerate AI model development timelines significantly. While programming challenges remain, widespread adoption of multicore AI silicon over the next 5 years will transform the datacenter AI landscape and enable new frontiers in deep learning like digital twins, synthetic data, and AI safety.
Segmental Insights
Type of AI Chipset Insights
GPU (Graphics Processing Unit) AI Chipsets dominated the Global Artificial Intelligence Chipset Market in 2022 and is expected to maintain its dominance during the forecast period. GPU AI Chipsets held the largest share of the global artificial intelligence chipset market in 2022. GPUs are massively parallel processors that can handle multiple tasks simultaneously. They have thousands of smaller, more efficient cores designed to handle multiple tasks concurrently. GPUs are highly effective in algorithms where processing of large blocks of data is done in parallel by breaking them into smaller sub-problems, like in deep learning and neural networks. Training deep learning models requires massive amounts of computational power for tasks like hyper-parameter tuning which is well suited for GPUs. Additionally, GPUs offer higher power efficiency and performance per dollar relative to CPUs or other chip types making them ideal for AI workloads. Major technology companies like NVIDIA, AMD and Intel among others have been investing heavily in developing powerful GPUs with dedicated Tensor Cores and high memory bandwidths to meet the growing need of AI training and inference. With the rise in AI applications across various industries like automotive, healthcare and more, the demand for powerful and cost-effective AI processors is expected to continue growing rapidly. This will drive the dominance of GPU AI chipsets in the global market over the forecast period.
Technology Insights
Deep learning dominated the Global Artificial Intelligence Chipset Market in 2022 based on technology and is expected to maintain its dominance during the forecast period. Deep learning is a branch of machine learning that utilizes neural networks with multiple hidden layers between the input and output layers to learn representations of data with multiple levels of abstraction. Deep learning algorithms are capable of learning complex patterns and correlations in large datasets without being explicitly programmed where to look for the relevant information. These algorithms are modeled after the human brain in designing and developing neural networks for deep learning which can learn in an unsupervised manner. Deep learning has revolutionized various domains like computer vision, natural language processing, robotics and more by achieving human-level performance in tasks like image recognition, speech recognition and machine translation. The availability of big data, low-cost GPUs and improved deep learning algorithms have made deep learning ubiquitous in recent years. Majority of AI chipsets developed by companies are optimized for deep learning workloads to accelerate the training of deep neural networks on massive datasets. As deep learning applications continue to grow exponentially across industries for advanced analytics, predictive modeling and automation, the demand for deep learning chipsets will continue to surge. Deep learning chipsets offer high performance and power efficiency compared to general purpose CPUs for deep learning tasks. Hence deep learning technology will dominate the artificial intelligence chipset market during the forecast period with its superior capabilities and widespread adoption across all industries
.
Regional Insights
North America dominated the Global Artificial Intelligence Chipset Market in 2022 based on region and is expected to maintain its dominance during the forecast period. The United States holds the majority share in the North American as well as global AI chipset market due to the strong presence of leading technology companies in the country. The US has the largest number of AI startups and heavy investments in AI research and development compared to other regions. It is a global leader in the development of AI technologies and associated chipsets with the presence of major AI chipset manufacturers like NVIDIA, Intel, AMD and Qualcomm based in the US. These companies have been investing billions of dollars in developing cutting edge AI processors and accelerators. Additionally, substantial government funding in AI research through DARPA, NASA and other agencies has created a conducive environment for AI innovation in the region. Wide adoption of AI across industries like healthcare, automotive, finance, retail and more has created a huge demand for AI-based products and services in North America. Abundant availability of data, computing power, skilled workforce and early adoption of advanced technologies have made North America the forerunner in commercializing AI. With continued technological advancements, increasing focus on AI applications and massive investments flowing into the AI space from private as well as public sectors, North America is expected to retain its leading position as the largest regional market for AI chipsets during the forecast period.
Recent Developments
- In 2022, NVIDIA launchedits new Hopper GPU architecture which delivers up to 3x higher performance thanthe previous generation for AI workloads. Hopper GPUs are aimed at acceleratingAI and high performance computing applications.
- Intel acquired TowerSemiconductor in 2022 to expand its foundry services and dedicated productioncapacity for Intel's advanced packaging technologies including its AI andgraphics products.
- Samsung unveiled its newCharm AI processor in 2022 which delivers over 260 trillion operations persecond (TOPS) of AI performance. It targets AI applications across mobile, IoT,automotive sectors.
- Qualcomm launched theSnapdragon 8 Gen 2 platform in 2022 featuring the company's 4th generation AIengine which delivers 4x faster AI performance than the previous generation.
- AMD launched its MI250 AIaccelerator card in 2022 powered by its CDNA 2 architecture. It delivers over3x better performance and power efficiency than the previous generation.
- Graphcore launched its newIPU-POD8I processor in 2022 delivering over 1 exaops of AI processing power. Ittargets AI training for NLP and computer vision workloads.
- Tenstorrent unveiled itsnew AI chip called Jupiter in 2022 which delivers over 100 teraops ofperformance targeting AI inference applications.
- Google acquired Mandiant in2022 to strengthen its cloud security offerings through Mandiant's expertise inincident response and cybersecurity services.
Key Market Players
- NVIDIA Corporation
- INTEL CORPORATION
- IBM Corporation
- Microsoft Corporation
- Amazon Web Services
- Qualcomm
- AlphabetInc
- SamsungElectronics Co. Ltd
- MicronTechnology, In
- Xilinx,Inc
By Type of AI Chipset | By Technology | By End-User Industry | By Region |
|
|
|
|
Related Reports
- Generative AI in Logistics Market - By Type (Variational Autoencoder (VAE), Generative Adversarial Networks (GANs), Recu...
- AI in Industrial Machinery Market - By Component (Hardware, Software, Services), By Technology (Machine Learning, Comput...
- Explainable AI Market Size - By Component (Solution, Service), By Software Service (Standalone Software, Integrated Soft...
- Casual AI Market Size - By Offering (Platform [ Deployment {Cloud, On-premises}], Services [Consulting, Deployment & Int...
- Metaverse for Automotive Market Size - By Component (Hardware, Software, Services), By Technology (Virtual Reality, Augm...
- SVOD Market Size - By Content (General Entertainment, Niche Content, Original Programming), By Pricing Model (Premium, A...
Table of Content
To get a detailed Table of content/ Table of Figures/ Methodology Please contact our sales person at ( chris@marketinsightsresearch.com )
List Tables Figures
To get a detailed Table of content/ Table of Figures/ Methodology Please contact our sales person at ( chris@marketinsightsresearch.com )
FAQ'S
For a single, multi and corporate client license, the report will be available in PDF format. Sample report would be given you in excel format. For more questions please contact:
Within 24 to 48 hrs.
You can contact Sales team (sales@marketinsightsresearch.com) and they will direct you on email
You can order a report by selecting payment methods, which is bank wire or online payment through any Debit/Credit card, Razor pay or PayPal.
Discounts are available.
Hard Copy