Artificial Intelligence in Asset Management Market – Global Industry Size, Share, Trends, Opportunity, and Forecast Segmented By Technology (Machine Learning, Natural Language Processing (NLP), Others), By Deployment Mode (On-Premises, Cloud), By Application (Portfolio Optimization, Conversational Platform, Risk & Compliance, Data Analysis, and Others), Region, By Competition, 2018-2028
Published Date: November - 2024 | Publisher: MIR | No of Pages: 320 | Industry: ICT | Format: Report available in PDF / Excel Format
View Details Buy Now 2890 Download Sample Ask for Discount Request CustomizationArtificial Intelligence in Asset Management Market – Global Industry Size, Share, Trends, Opportunity, and Forecast Segmented By Technology (Machine Learning, Natural Language Processing (NLP), Others), By Deployment Mode (On-Premises, Cloud), By Application (Portfolio Optimization, Conversational Platform, Risk & Compliance, Data Analysis, and Others), Region, By Competition, 2018-2028
Forecast Period | 2024-2028 |
Market Size (2022) | USD 2.58 billion |
CAGR (2023-2028) | 35.67% |
Fastest Growing Segment | Machine Learning |
Largest Market | North America |
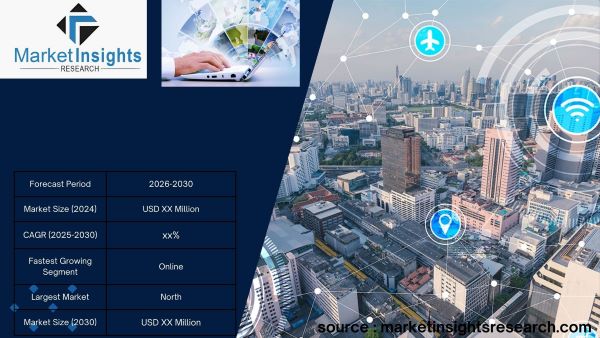
Market Overview
Global Artificial Intelligence in Asset Management Market has experienced tremendous growth in recent years and is poised to continue its strong expansion. The Artificial Intelligence in Asset Management Market reached a value of USD 2.58 billion in 2022 and is projected to maintain a compound annual growth rate of 35.67% through 2028.
Key Market Drivers
Enhanced Investment Decision-Making with AI
One of the primary drivers propelling the Global Artificial Intelligence in Asset Management Market is the significant enhancement it brings to investment decision-making processes. AI-driven solutions empower asset managers and investors with unprecedented capabilities to analyze vast and complex datasets in real-time. These AI algorithms can process news articles, financial reports, social media sentiment, and historical market data to identify patterns, trends, and investment opportunities that might be difficult for human analysts to discern.
By leveraging machine learning and natural language processing (NLP) techniques, AI models can extract valuable insights from unstructured data sources, such as news articles and social media posts. This enables asset managers to make more informed and timely investment decisions, anticipate market movements, and adjust portfolios dynamically to maximize returns while minimizing risks. Additionally, AI-powered predictive analytics provide asset managers with tools to assess asset performance, detect anomalies, and anticipate market fluctuations. This proactive approach allows for better risk management and improved investment strategies, ultimately leading to superior financial outcomes for both asset managers and their clients. In summary, the application of AI in asset management is driven by the quest for more precise and data-driven investment decisions. The ability of AI to analyze vast amounts of data, identify patterns, and provide actionable insights empowers asset managers to optimize their investment strategies and deliver better results for their clients..
In summary, digital transformation initiatives are a primary driver of the global Artificial Intelligence in Asset Management market. Organizations are turning to software consultants to help them navigate the complexities of modernizing their IT infrastructure, leveraging data analytics, and achieving seamless integration.
Increasing Complexity of Financial Markets
The Global Artificial Intelligence in Asset Management Market is also driven by the increasing complexity of financial markets. In today's interconnected global economy, financial markets are influenced by a multitude of factors, including geopolitical events, economic indicators, and rapidly changing investor sentiments. This complexity creates a challenging environment for asset managers and investors to navigate effectively.
AI solutions offer a competitive advantage in this complex landscape by providing the ability to process and interpret large volumes of data rapidly. They can assess the potential impact of various factors on asset prices, helping asset managers make more informed decisions in real-time. AI can analyze historical market data and identify patterns that indicate potential market movements or opportunities.
Moreover, the integration of AI-driven risk management tools enables asset managers to better understand and mitigate risks associated with their portfolios. By continuously monitoring the market and assessing portfolio vulnerabilities, AI systems can alert asset managers to potential threats and suggest risk mitigation strategies. The complexity of financial markets is expected to continue growing, making AI-driven asset management solutions increasingly essential for staying competitive and achieving positive investment outcomes. Therefore, the adoption of AI in asset management is driven by the need for advanced tools to navigate the intricate and ever-changing financial landscape effectively.
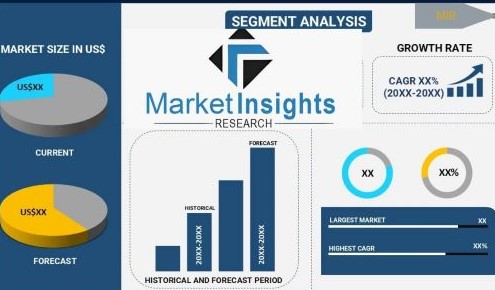
Demand for Personalized Investment Strategies
Another significant driver for the Global Artificial Intelligence in Asset Management Market is the increasing demand for personalized investment strategies. Traditional one-size-fits-all investment approaches no longer meet the expectations of today's investors. Instead, individuals and institutional clients seek customized investment solutions tailored to their unique financial goals, risk tolerance, and preferences. AI-driven asset management platforms are well-suited to address this demand for personalization. These platforms leverage AI algorithms to analyze clients' financial profiles, investment objectives, and risk preferences. They can then generate personalized investment strategies that align with each client's specific goals. Additionally, AI enables continuous monitoring and adjustment of portfolios to ensure they remain in line with clients' evolving needs and market conditions. By providing personalized recommendations and adapting to changing circumstances, AI-driven asset management platforms enhance customer satisfaction, loyalty, and retention.
In conclusion, the growing demand for personalized investment strategies is a significant driver pushing the adoption of AI in asset management. AI's ability to create tailored investment solutions and continuously adapt to individual client needs positions it as a critical tool for asset managers seeking to attract and retain clients in a competitive market. This driver highlights the transformative potential of AI in reshaping the asset management industry to better serve the diverse needs of investors.
Key Market Challenges
Data Quality and Availability
One of the foremost challenges in the Global Artificial Intelligence in Asset Management Market is the quality and availability of data. AI-driven asset management heavily relies on data for investment decision-making, risk assessment, and portfolio optimization. However, the financial data landscape is often fragmented, with information scattered across various sources such as market feeds, economic indicators, company reports, and alternative data streams like social media sentiment. This fragmentation makes it challenging to consolidate and integrate data efficiently into AI models. Furthermore, the accuracy and reliability of data sources are critical. Inaccurate or outdated data can lead to erroneous investment decisions, increased risks, and potential losses. Moreover, asset managers must grapple with data privacy and compliance issues, especially in the era of stringent regulations like GDPR and Dodd-Frank. Handling sensitive financial information while adhering to these regulations requires robust data management practices and compliance measures. Additionally, the use of alternative data sources, such as satellite imagery or web scraping, introduces complexities due to unstructured, noisy, or biased data that need careful handling and preprocessing.
Model Interpretability and Explainability
Another significant challenge in the Global Artificial Intelligence in Asset Management Market pertains to the interpretability and explainability of AI models. As AI algorithms become more advanced and complex, they often operate as "black-box" models. This means that while these models can make accurate predictions and investment decisions, the inner workings of these models are not readily interpretable by humans. This opacity can raise concerns among asset managers as they may struggle to explain investment decisions to clients or regulators, particularly when regulatory bodies like the SEC require transparency and explanations for investment strategies. Ensuring model interpretability and explainability is not only a regulatory necessity but also essential for effective risk management. Understanding the risk factors and drivers behind AI-driven investment decisions is crucial for mitigating risks and maintaining portfolio stability.
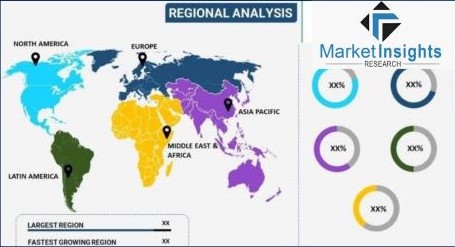
Overfitting and Model Robustness
Overfitting and model robustness are persistent challenges in the Global Artificial Intelligence in Asset Management Market. Overfitting occurs when an AI model performs exceptionally well on training data but struggles to generalize to new, unseen data, leading to suboptimal performance in real-world scenarios. Model robustness, on the other hand, relates to the ability of AI models to adapt and perform well in changing market conditions. Given the dynamic nature of financial markets with abrupt changes and shifting patterns, AI models that are overfit to historical data may not respond effectively to new market conditions. During extreme events such as market crashes or economic crises, data scarcity can also pose a significant challenge. AI models that have not encountered similar events during training may struggle to provide meaningful insights or predictions during these periods. To address these challenges, asset managers must invest in ongoing model monitoring, validation, and retraining to ensure that AI models remain robust, well-calibrated, and capable of handling various market scenarios. Regulatory bodies are increasingly scrutinizing AI-driven models in asset management, further emphasizing the importance of model robustness and compliance.
In conclusion, the Global Artificial Intelligence in Asset Management Market faces significant challenges related to data quality and availability, model interpretability and explainability, and overfitting/model robustness. These challenges underscore the complexity of integrating AI into asset management strategies and the importance of addressing them to harness the full potential of AI while managing risks effectively.
Key Market Trends
Explainable AI (XAI) for Transparency and Compliance
One prominent trend in the Global Artificial Intelligence in Asset Management Market is the increasing adoption of Explainable AI (XAI) to enhance transparency and ensure compliance with regulatory requirements. XAI refers to AI systems designed to provide human-understandable explanations for their decisions and predictions, addressing the challenge of black-box AI models. In asset management, where regulatory scrutiny is on the rise, ensuring that AI-driven investment decisions can be explained and justified is crucial. XAI technologies are becoming integral to asset management AI platforms, allowing asset managers to understand and communicate the reasoning behind AI-generated investment strategies. This trend is particularly relevant given the regulatory mandates in the financial industry that necessitate transparency and accountability. For instance, the SEC's Regulation Best Interest (Reg BI) in the United States requires financial advisors to act in the best interest of clients and provide clear disclosures regarding investment decisions. Asset managers are leveraging XAI to bridge the gap between complex AI models and human understanding. With XAI, they can dissect the decision-making process of AI algorithms, highlighting the key factors, variables, and data points that influence investment choices. This not only aids in meeting regulatory requirements but also builds trust with clients who seek to comprehend the rationale behind their investment recommendations.
Moreover, XAI enhances risk management by allowing asset managers to identify potential biases or anomalies in AI models. This proactive approach helps mitigate risks associated with AI-driven decision-making, ensuring that investment strategies remain in line with regulatory standards and clients' best interests.As regulatory scrutiny continues to evolve, the incorporation of XAI in asset management AI solutions will be a pivotal trend, enabling asset managers to navigate compliance challenges and foster trust with clients and regulatory authorities alike.
ESG Integration for Sustainable Investing
Environmental, Social, and Governance (ESG) considerations are rapidly emerging as a dominant trend in the Global Artificial Intelligence in Asset Management Market. ESG factors encompass a range of criteria related to a company's environmental impact, social responsibility, and corporate governance practices. Asset managers are increasingly incorporating ESG data and principles into their investment strategies to meet growing demand for sustainable and responsible investing. AI plays a critical role in ESG integration by enabling asset managers to analyze vast datasets and assess the ESG performance of companies and assets. AI algorithms can comb through news articles, financial reports, social media sentiment, and other sources to evaluate how ESG factors impact investment opportunities and risks. This comprehensive analysis assists asset managers in making informed decisions aligned with ESG goals. The integration of ESG into AI-driven asset management is driven by both investor demand and regulatory changes. Investors are increasingly seeking investments that align with their values, leading to a surge in ESG-focused funds. Regulatory bodies in various regions are also implementing disclosure requirements related to ESG factors, compelling asset managers to consider ESG in their investment processes. Furthermore, AI-driven ESG analysis enables asset managers to uncover valuable insights that may not be apparent through traditional methods. This includes identifying companies with strong ESG practices that are likely to outperform their peers in the long run or detecting ESG-related risks that could impact the performance of investments.
As the emphasis on sustainability and responsible investing continues to grow, the integration of ESG factors into AI-driven asset management strategies is expected to be a transformative trend, reshaping investment decision-making and aligning portfolios with environmental and social goals.
Customized AI Solutions for Boutique Asset Managers
In the Global Artificial Intelligence in Asset Management Market, there is a notable trend towards the development of customized AI solutions tailored to the specific needs of boutique asset managers. While large asset management firms have the resources to invest in comprehensive AI platforms, smaller boutique firms are seeking ways to harness the power of AI without the scale of their larger counterparts. Customized AI solutions are designed to address the unique challenges and investment strategies of boutique asset managers. These solutions can range from AI-powered portfolio optimization tools to risk assessment platforms and AI-driven market sentiment analysis.
The trend towards customized AI solutions reflects the recognition that AI is not a one-size-fits-all solution. Boutique asset managers may have specialized investment niches, risk tolerance profiles, or client preferences that require tailored AI capabilities. By partnering with AI solution providers that offer customization options, boutique asset managers can access cutting-edge technology that aligns with their specific investment goals. Moreover, these customized AI solutions are often more cost-effective for boutique firms, as they can focus on the specific functionalities they need, avoiding unnecessary expenses associated with large-scale AI implementations. This democratization of AI technology allows boutique asset managers to compete effectively in a market where data-driven decision-making is increasingly essential.
As AI continues to evolve, the trend of customized solutions for boutique asset managers is likely to expand, enabling a broader range of firms to leverage the benefits of AI in enhancing their investment strategies and client services.
Segmental Insights
Technology Insights
The Machine Learning (ML) segment is dominating in the global artificial intelligence in asset management market.
Machine learning is a type of artificial intelligence that allows computers to learn without being explicitly programmed. ML algorithms are used to analyze large amounts of data and to identify patterns and trends. This information can then be used to make predictions and decisions.
ML is being used in asset management in a number of ways, includingPortfolio constructionML algorithms can be used to identify and select assets that are likely to perform well, based on historical data and other factors.
Risk managementML algorithms can be used to identify and assess risks associated with different investments.
Performance analysisML algorithms can be used to analyze portfolio performance and to identify areas for improvement.The growth of the ML segment is being driven by a number of factors, including
The increasing availability of dataAs more and more data is generated, ML algorithms are becoming more accurate and powerful.The decreasing cost of computing powerThe decreasing cost of computing power is making it more feasible to run ML algorithms on large datasets.The growing demand for asset management solutionsThe growing demand for asset management solutions is driving the demand for ML-based asset management solutions.Some of the major players in the ML segment of the global artificial intelligence in asset management market include IBM, SAS, and BlackRock.
The Natural Language Processing (NLP) segment is also a significant market for artificial intelligence in asset management. NLP algorithms are used to process and understand human language. This information can then be used to generate reports, provide insights to investors, and make investment decisions. The growth of the NLP segment is being driven by a number of factors, including
The increasing availability of unstructured dataAs more and more data is generated, more and more of it is unstructured. NLP algorithms can be used to process and understand unstructured data, which can provide valuable insights to asset managers.
The growing demand for insights from social media dataSocial media data can be a valuable source of insights for asset managers. NLP algorithms can be used to process and understand social media data, which can help asset managers to identify trends and make better investment decisions.
Some of the major players in the NLP segment of the global artificial intelligence in asset management market include Kensho and Sentient Technologies.
The Other segment includes a variety of other artificial intelligence technologies that are being used in asset management, such as computer vision and deep learning. These technologies are being used to develop new asset management solutions and to improve existing solutions.
This growth is being driven by a number of factors, including the increasing availability of data, the decreasing cost of computing power, and the growing demand for asset management solutions.
Download Free Sample Report
Regional Insights
North America is the dominating region in the global artificial intelligence in asset management market. .The growth of the artificial intelligence in asset management market in North America is being driven by a number of factors, including
High concentration of asset management firmsNorth America is home to a large number of asset management firms, which are major consumers of artificial intelligence in asset management solutions.
Early adoption of new technologiesNorth American asset management firms are typically early adopters of new technologies, which drives the demand for artificial intelligence in asset management solutions to help them improve their investment performance and reduce risk.Presence of leading artificial intelligence in asset management vendorsNorth America is home to some of the world's leading artificial intelligence in asset management vendors, such as IBM, SAS, and BlackRock.
Other key regions in the global artificial intelligence in asset management market include
EuropeThis region is also a major market for artificial intelligence in asset management, with a number of large multinational asset management firms headquartered in the region.
Asia PacificThis region is expected to grow at the fastest CAGR during the forecast period, driven by the increasing adoption of artificial intelligence in asset management by asset management firms in the region.Middle East and AfricaThis region is expected to grow at a moderate CAGR during the forecast period, driven by the increasing government investments in IT infrastructure and the growing adoption of artificial intelligence in asset management by asset management firms in the region.This growth is being driven by a number of factors, including the increasing availability of data, the decreasing cost of computing power, and the growing demand for asset management solution.
Recent Developments
- IBM has launched a newsuite of artificial intelligence (AI) solutions for asset management. Thesesolutions will help asset managers to improve their investment performance,reduce risk, and automate their operations.
- SAS has partnered withGoogle Cloud to launch a new cloud-based platform for artificial intelligencein asset management. This platform will help asset managers to accelerate theirdigital transformation and to improve their operational efficiency.
Key Market Players
- BlackRock, Inc.
- State Street Corporation
- Bridgewater Associates
- Two Sigma Investments
- AQR Capital Management
- Invesco Ltd.
- Schroders plc
- Vanguard Group
- Goldman Sachs Asset Management
- Morgan Stanley Investment Management
By Technology | By Deployment Mode | By Application | By Region |
|
|
|
|
Related Reports
- SVOD Market Size - By Content (General Entertainment, Niche Content, Original Programming), By Pricing Model (Premium, A...
- Multimodal AI Market Size - By Component (Solution, Service), By Technology (Machine Learning, Natural Language Processi...
- AVOD Market Size - By Content (Movies, TV Shows, Original Series, News, Sports, User-generated Content), By Platform (S...
- Premium Finance Market - By Type (Life Insurance, Non-life Insurance), By Interest Rate (Fixed Interest Rate, Floating I...
- Gift Cards Market - By Type (Closed-loop Gift Cards, Open-loop Gift Cards), By Distribution Channel (Online, Offline), B...
- Biometric Payment Market Size - By Biometric Mode (Fingerprint Recognition, Facial Recognition, Voice Recognition, Iris ...
Table of Content
To get a detailed Table of content/ Table of Figures/ Methodology Please contact our sales person at ( chris@marketinsightsresearch.com )
List Tables Figures
To get a detailed Table of content/ Table of Figures/ Methodology Please contact our sales person at ( chris@marketinsightsresearch.com )
FAQ'S
For a single, multi and corporate client license, the report will be available in PDF format. Sample report would be given you in excel format. For more questions please contact:
Within 24 to 48 hrs.
You can contact Sales team (sales@marketinsightsresearch.com) and they will direct you on email
You can order a report by selecting payment methods, which is bank wire or online payment through any Debit/Credit card, Razor pay or PayPal.
Discounts are available.
Hard Copy