Reinforcement Learning Market – Global Industry Size, Share, Trends, Opportunity, and Forecast, Segmented By Deployment (On-Premises, Cloud based), By Enterprise size (Large, Small & Medium Enterprises), By End-user (Healthcare, BFSI, Retail, Telecommunication, Government & Defense, Energy & Utilities, Manufacturing), By Region, and By Competition, 2018-2028
Published Date: November - 2024 | Publisher: MIR | No of Pages: 320 | Industry: ICT | Format: Report available in PDF / Excel Format
View Details Buy Now 2890 Download Sample Ask for Discount Request CustomizationReinforcement Learning Market – Global Industry Size, Share, Trends, Opportunity, and Forecast, Segmented By Deployment (On-Premises, Cloud based), By Enterprise size (Large, Small & Medium Enterprises), By End-user (Healthcare, BFSI, Retail, Telecommunication, Government & Defense, Energy & Utilities, Manufacturing), By Region, and By Competition, 2018-2028
Forecast Period | 2024-2028 |
Market Size (2022) | USD 8.12 Billion |
CAGR (2023-2028) | 21.33% |
Fastest Growing Segment | Small & Medium Enterprises |
Largest Market | North America |
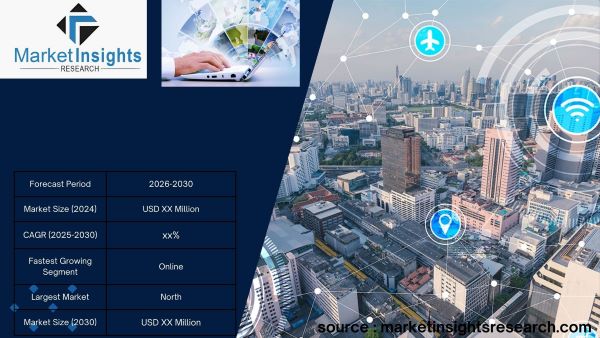
Market Overview
The global reinforcement learning (RL) market has been steadily expanding as organizations across various industries recognize the transformative potential of RL algorithms. RL, a subset of machine learning, enables systems to learn and make intelligent decisions through trial and error, mimicking human learning processes. This technology has found applications in diverse sectors, ranging from healthcare and finance to manufacturing and telecommunications.
One of the primary drivers of the RL market's growth is the ability to solve complex decision-making problems. In healthcare, RL is revolutionizing personalized medicine, clinical decision support, and drug discovery, leading to more effective treatments and improved patient outcomes. In the financial sector, RL powers algorithmic trading and fraud detection systems, enhancing risk management and profit generation. In manufacturing, RL optimizes processes, predictive maintenance, and quality control, driving operational efficiency.
Moreover, the RL market benefits from advancements in computing power and data availability, allowing organizations to train more sophisticated RL models. Cloud-based RL solutions have made these technologies more accessible to businesses of all sizes. As a result, small and medium-sized enterprises (SMEs) are increasingly adopting RL to gain a competitive edge.
While North America currently dominates the global RL market due to its thriving tech ecosystem and early adoption, other regions like Europe and Asia-Pacific are witnessing rapid growth. In the coming years, the RL market is poised for significant expansion as industries continue to explore innovative applications, and vendors develop more user-friendly RL solutions to cater to a broader range of businesses. The market's evolution promises to revolutionize decision-making processes across multiple sectors, further enhancing efficiency, cost-effectiveness, and competitiveness for organizations worldwide.
Key Market Drivers
Rapid Advancements in Deep Learning and Neural Networks
Deep learning techniques, particularly deep neural networks, have played a pivotal role in the resurgence of Reinforcement Learning. These architectures enable RL algorithms to handle high-dimensional data, leading to breakthroughs in applications such as game playing, robotics, and autonomous vehicles. The continuous development and refinement of deep learning methods are driving the adoption of RL across industries.
Emerging Applications in Autonomous Systems
Reinforcement Learning is finding extensive applications in autonomous systems, including self-driving cars, drones, and robotics. As the demand for autonomous technologies grows, so does the need for RL algorithms that can enable these systems to learn and adapt to complex environments. The potential for improved safety, efficiency, and decision-making in autonomous systems is a significant driver in the RL market.
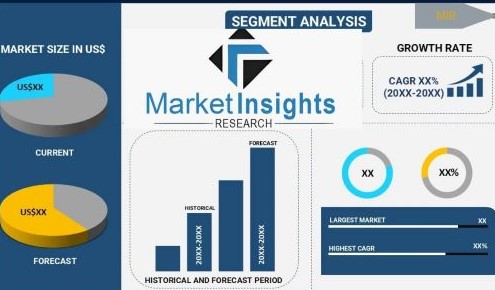
AI in Healthcare and Drug Discovery
Healthcare and pharmaceutical industries are increasingly utilizing Reinforcement Learning for drug discovery, personalized medicine, and disease diagnosis. RL models can optimize drug candidate selection and clinical trial designs, reducing costs and accelerating the development of new therapies. This promising application is driving investments and research in RL for healthcare.
Enhanced Natural Language Processing (NLP)
Reinforcement Learning is contributing to advancements in Natural Language Processing, enabling machines to understand and generate human-like text. Chatbots, virtual assistants, and automated content generation benefit from RL algorithms that can optimize language generation and interaction. The demand for improved NLP capabilities is propelling the adoption of RL in this domain.
Gaming and Entertainment Industry
The gaming and entertainment sector has been an early adopter of Reinforcement Learning, with notable successes in game playing, including AlphaGo and OpenAI's GPT models. This trend is expected to continue as gaming companies seek to enhance player experiences, create more challenging opponents, and develop content with AI-generated narratives. The gaming industry's support and investment in RL research are fostering innovation.
Energy Management and Sustainability
In the pursuit of sustainable energy solutions, RL is being applied to optimize energy consumption, grid management, and renewable energy sources. RL algorithms can control and manage energy resources more efficiently, reduce carbon footprints, and enhance energy grid resilience, making them crucial drivers in the push for sustainability.
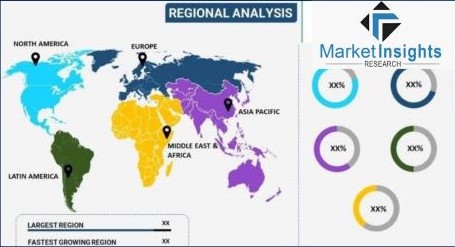
Finance and Trading Algorithms
Financial institutions are increasingly using Reinforcement Learning for algorithmic trading, portfolio optimization, and risk management. RL's ability to learn from historical data and adapt to changing market conditions can provide a competitive advantage in financial markets.
Cross-Industry Collaboration and Open Source Frameworks
Collaborative efforts among academia, industry, and open-source communities have led to the development of RL frameworks and libraries that facilitate research and application development. OpenAI's Gym and TensorFlow's RL libraries, for instance, have democratized access to RL tools, fostering innovation and adoption.
Key Market Challenges
Data Efficiency and Sample Complexity
Reinforcement Learning often requires a substantial amount of data and interactions with an environment to learn effective policies. This high sample complexity can be a significant challenge, especially in real-world applications where collecting data can be costly or time-consuming.
Lack of Interpretability and Explainability
Many RL algorithms, especially deep reinforcement learning models, lack interpretability and explainability. Understanding why a particular decision or policy is chosen by an RL agent is crucial, especially in applications like healthcare or finance, where transparency and accountability are essential.
Safety and Ethical Concerns
Ensuring the safety of RL-driven systems, such as autonomous vehicles or robotics, is a major challenge. RL algorithms may learn unsafe policies during the training process, and there's a need for techniques to guarantee safe behavior and address ethical concerns associated with RL applications.
Sample Efficiency in Continuous Control Tasks
In continuous control tasks, where actions are not discrete but can take on a range of values, RL algorithms often struggle with sample efficiency. Training an RL agent to perform well in such tasks may require a large number of interactions with the environment, making it impractical in some scenarios.
Generalization and Transfer Learning
Generalizing knowledge learned in one environment to another (transfer learning) and adapting to new, unseen situations are challenges in RL. RL models often struggle with generalization, which is crucial for practical applications that involve dynamic and changing environments.
Key Market Trends
Increasing Adoption Across Industries
Reinforcement Learning (RL) is gaining traction in various industries, including finance, healthcare, robotics, and autonomous systems. Organizations are realizing the potential of RL to optimize decision-making processes, enhance automation, and improve overall efficiency.
Advancements in Deep Reinforcement Learning (DRL)
Deep Reinforcement Learning, which combines deep learning with RL algorithms, is witnessing significant advancements. DRL has achieved remarkable results in complex tasks like game playing and autonomous navigation. As DRL techniques mature, they are finding applications in real-world scenarios.
Development of RL Frameworks and Tools
The development of user-friendly RL frameworks and tools is simplifying the adoption of RL technology. Open-source libraries like TensorFlow and PyTorch offer RL libraries, making it easier for researchers and developers to experiment and implement RL algorithms.
AI-driven Personalization and Recommendation Systems
In the e-commerce and content streaming sectors, RL is being used to enhance recommendation systems. These systems are becoming more personalized, resulting in improved customer engagement and satisfaction. RL algorithms enable platforms to optimize content delivery and product recommendations based on user preferences.
Autonomous Vehicles and Robotics
The automotive and robotics industries are increasingly integrating RL for autonomous navigation and decision-making. RL algorithms help vehicles and robots learn from their interactions with the environment, leading to safer and more efficient autonomous systems.
Segmental Insights
Deployment Insights
On-Premises segment
However, the on-premises RL segment faced challenges related to scalability and maintenance costs. Implementing and managing on-premises hardware and software can be resource-intensive and scaling up to meet growing demands often required significant investments.
Enterprise size Insights
Large Enterprises segment
Resource AllocationLarge enterprises typically have more substantial financial resources to invest in RL research and development. They can allocate significant budgets to hire data scientists, AI engineers, and researchers dedicated to RL projects.
Complex Use CasesLarge enterprises often deal with complex business challenges that can benefit from RL applications. Industries such as finance, healthcare, autonomous vehicles, and industrial automation have adopted RL to optimize operations, enhance decision-making, and drive innovation.
Data AvailabilityLarge enterprises generate vast volumes of data, which are essential for training RL algorithms effectively. They have extensive datasets that can be used to fine-tune RL models for specific tasks.
InfrastructureScaling RL solutions requires substantial computing power, which large enterprises can afford. They can leverage cloud resources or build on-premises infrastructure to support RL training and deployment.
Regulatory ComplianceCertain industries, like finance and healthcare, have stringent regulatory requirements. Large enterprises often have the resources and expertise to navigate complex compliance and security standards associated with RL implementations.
Regional Insights
North America
North America boasts a large pool of skilled professionals in artificial intelligence (AI) and machine learning (ML). The region's universities produce a steady stream of talented graduates, and its diverse workforce includes experts from around the world. This talent pool is critical for the development and implementation of RL solutions.
North America has a vibrant startup ecosystem, particularly in tech hubs like Silicon Valley and Boston. Many RL startups have emerged in these regions, focusing on various applications such as autonomous vehicles, robotics, healthcare, and finance. Access to venture capital funding and mentorship has accelerated the growth of these startups.
North American industries, including finance, healthcare, gaming, and autonomous systems, have been early adopters of RL technology. For example, major financial institutions use RL for algorithmic trading and risk management, while healthcare companies employ it in drug discovery and personalized medicine. This adoption has created a strong demand for RL solutions.
Recent Developments
In June 2020, Mitsubishi Electric Corporation announced that it has developed a cooperative artificial intelligence (AI) technology that enhances work collaboration between humans and machines by using inverse reinforcement learning (IRL) to learn and imitate the actions of skilled workers. IRL, one of the key features of Mitsubishi Electric's Maisart AI technology, enables machines to imitate human-like actions based on relatively small amounts of data. The new cooperative AI technology will be further refined through test deployment in automated guided vehicles (AGVs) and robots at production and distribution sites where machines operate alongside humans. Eventually, the technology is expected to be used in autonomous driving vehicles and other applications.
Key Market Players
- SAP SE
- IBM Corporation
- Amazon Web Services, Inc.
- SAS Institute Inc.
- Baidu, Inc.
- RapidMiner
- Cloud Software Group, Inc.
- Intel Corporation
- NVIDIA Corporation
- Hewlett Packard EnterpriseDevelopment LP
By Deployment | By Enterprise size | By End-user | By Region |
On-Premises Cloud based | Large Small & Medium Enterprises | Healthcare BFSI Retail Telecommunication Government & Defense Energy & Utilities Manufacturing | North America Europe South America Middle East & Africa Asia Pacific |
Related Reports
- Cognitive Computing Market Size - By Technology (Machine Learning, Natural Language Processing (NLP), Human Computer Int...
- Cognitive Computing Market Size - By Technology (Machine Learning, Natural Language Processing (NLP), Human Computer In...
- Decentralized Identity Market Size, By Identity Type (Biometrics, Non-Biometrics), By Enterprise Size (Large Enterprise,...
- Blockchain Identity Management Market Size - By Offering (Software, Service), By Provider Type (Application Provider, Mi...
- PayTV Market - By Technology (Cable TV, Satellite TV, Internet Protocol TV), By Subscription Type (Postpaid, Prepaid), ...
- Generative AI in Logistics Market - By Type (Variational Autoencoder (VAE), Generative Adversarial Networks (GANs), Recu...
Table of Content
To get a detailed Table of content/ Table of Figures/ Methodology Please contact our sales person at ( chris@marketinsightsresearch.com )
List Tables Figures
To get a detailed Table of content/ Table of Figures/ Methodology Please contact our sales person at ( chris@marketinsightsresearch.com )
FAQ'S
For a single, multi and corporate client license, the report will be available in PDF format. Sample report would be given you in excel format. For more questions please contact:
Within 24 to 48 hrs.
You can contact Sales team (sales@marketinsightsresearch.com) and they will direct you on email
You can order a report by selecting payment methods, which is bank wire or online payment through any Debit/Credit card, Razor pay or PayPal.
Discounts are available.
Hard Copy