Self-Learning Neuromorphic Chip Market – Global Industry Size, Share, Trends, Opportunity, and Forecast, Segmented By Vertical (Power & Energy, Media & Entertainment, Smartphones, Healthcare, Automotive, Consumer Electronics, Aerospace, Defense), By Application (Data Mining, Signal Recognition, Image Recognition), By Region, By Competition, 2019-2029
Published Date: November - 2024 | Publisher: MIR | No of Pages: 320 | Industry: ICT | Format: Report available in PDF / Excel Format
View Details Buy Now 2890 Download Sample Ask for Discount Request CustomizationForecast Period | 2025-2029 |
Market Size (2023) | USD 861 Million |
CAGR (2024-2029) | 19.1% |
Fastest Growing Segment | Image Recognition |
Largest Market | North America |
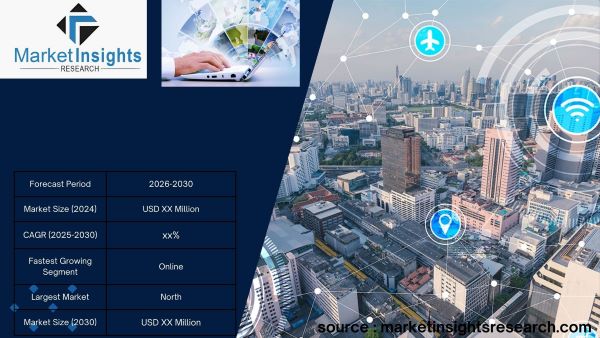
Market Overview
Global Self-Learning Neuromorphic Market was valued at USD 861 Million in 2023 and is anticipated to project robust growth in the forecast period with a CAGR of 19.1% through 2029. The Global Self-Learning Neuromorphic Market is experiencing significant growth propelled by the escalating demand for artificial intelligence (AI) solutions across diverse sectors. Neuromorphic computing, inspired by the human brain's neural networks, is revolutionizing the AI landscape. This technology enables machines to learn and make decisions autonomously, fostering unparalleled advancements in robotics, healthcare, automotive, and electronics industries. The rising need for intelligent systems capable of processing vast datasets in real-time, coupled with the pursuit of energy-efficient computing solutions, has catapulted the adoption of self-learning neuromorphic platforms. Moreover, the market is witnessing substantial investments in research and development, driving the innovation of more sophisticated neuromorphic hardware and software. Companies are leveraging these advancements to enhance their products and services, leading to increased efficiency, improved customer experiences, and competitive advantages. With ongoing technological advancements and a growing emphasis on AI-driven solutions, the Global Self-Learning Neuromorphic Market is poised for sustained expansion, transforming industries and reshaping the future of intelligent computing.
Key Market Drivers
Rising Demand for Artificial Intelligence Solutions
The Global Self-Learning Neuromorphic Market is driven by the soaring demand for artificial intelligence (AI) solutions across various industries. As businesses increasingly recognize the transformative potential of AI technologies, the market for self-learning neuromorphic systems has witnessed unprecedented growth. Companies are deploying these advanced computing platforms to enhance their operational efficiency, automate complex tasks, and gain valuable insights from vast datasets. The ability of self-learning neuromorphic systems to mimic the human brain's learning processes offers a unique advantage, enabling machines to adapt and improve their performance over time. In sectors such as healthcare, finance, and manufacturing, the demand for AI-powered solutions is particularly high, driving the adoption of self-learning neuromorphic technologies. Moreover, the proliferation of Internet of Things (IoT) devices and the need for real-time data processing have further accelerated the integration of self-learning neuromorphic systems, making them indispensable components of modern AI ecosystems. This increasing reliance on AI-driven capabilities is propelling the Global Self-Learning Neuromorphic Market into a new era of innovation and technological advancement.
Advancements in Neuromorphic Hardware and Software
Another significant driver fueling the growth of the Global Self-Learning Neuromorphic Market is the continuous advancements in neuromorphic hardware and software. Researchers and technology companies are investing heavily in developing more sophisticated and efficient neuromorphic chips, which form the backbone of self-learning systems. These chips are designed to process information in a manner akin to the human brain, enabling faster and more accurate computations. Additionally, there have been remarkable strides in neuromorphic software algorithms, allowing for the creation of complex neural networks and enhancing the learning capabilities of machines. The synergy between cutting-edge hardware and intelligent software algorithms has unlocked new possibilities in AI applications, ranging from natural language processing and image recognition to autonomous robotics. As these advancements continue to evolve, the Global Self-Learning Neuromorphic Market is experiencing a surge in demand from industries seeking innovative solutions to complex challenges, driving the market forward.
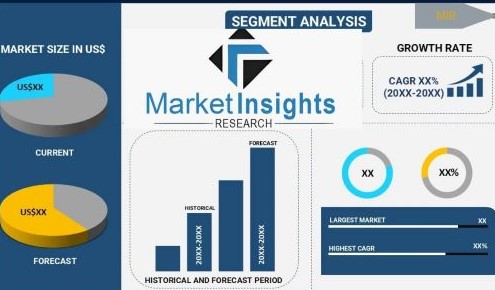
Energy-Efficient Computing Solutions
Energy efficiency has become a paramount concern in the field of computing, especially as the demand for powerful AI solutions rises. Traditional computing architectures often consume significant amounts of energy, leading to higher operational costs and environmental impact. In contrast, self-learning neuromorphic systems are inherently energy-efficient, mirroring the brain's ability to process information using minimal power. This unique characteristic makes them highly attractive for applications where power consumption is a critical consideration, such as in portable devices, IoT sensors, and autonomous vehicles. The ability of self-learning neuromorphic systems to deliver exceptional computational capabilities while conserving energy addresses a crucial need in the market. Industries seeking sustainable and eco-friendly computing solutions are increasingly turning to self-learning neuromorphic technologies, thereby driving the market's growth and fostering a greener approach to advanced computing.
Research and Development Investments
The Global Self-Learning Neuromorphic Market is bolstered by substantial investments in research and development (R&D) activities. Leading technology companies, academic institutions, and government organizations are dedicating significant resources to furthering the understanding of neuromorphic computing and advancing its applications. These investments support fundamental research in neuroscience, material science, and computer engineering, driving the development of novel neuromorphic hardware architectures and intelligent algorithms. R&D efforts are focused on overcoming existing limitations, such as scalability and complexity, to create more efficient and reliable self-learning systems. Collaborative initiatives between researchers and industry players have resulted in breakthrough innovations, propelling the market's growth trajectory. The continuous influx of funding into R&D initiatives ensures that the Global Self-Learning Neuromorphic Market remains at the forefront of technological innovation, offering businesses and consumers cutting-edge solutions that transform the way they interact with AI technologies.
Diverse Industry Applications
The versatility of self-learning neuromorphic systems in addressing a wide array of industry challenges serves as a compelling driver for market expansion. These systems find applications in diverse sectors, including healthcare, automotive, finance, manufacturing, and telecommunications. In healthcare, self-learning neuromorphic technologies are utilized for complex medical diagnoses, drug discovery, and personalized treatment plans. The automotive industry leverages these systems for the development of autonomous vehicles, enabling them to perceive their surroundings and make real-time decisions. Financial institutions deploy self-learning neuromorphic algorithms to detect fraudulent activities and optimize trading strategies. Additionally, in manufacturing, these systems enhance predictive maintenance, improving operational efficiency and reducing downtime. The adaptability of self-learning neuromorphic technologies to different industry requirements positions them as indispensable tools for innovation and problem-solving. As businesses across various sectors recognize the potential of these technologies to revolutionize their operations, the Global Self-Learning Neuromorphic Market continues to witness widespread adoption, driving its sustained growth and impact on diverse industries.
Key Market Challenges
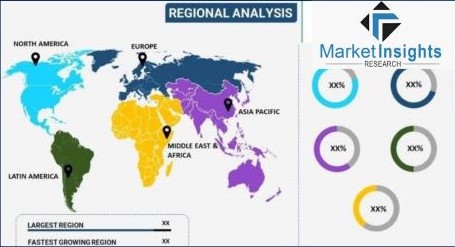
Complexity of Neuromorphic System Integration
One of the significant challenges facing the Global Self-Learning Neuromorphic Market is the complexity associated with integrating neuromorphic systems into existing technological infrastructures. Neuromorphic computing, designed to replicate the intricate neural networks of the human brain, involves highly complex algorithms and hardware configurations. Integrating these systems seamlessly with conventional computing technologies often proves challenging. Compatibility issues, data synchronization problems, and the need for specialized expertise in both neuromorphic and traditional computing domains pose substantial hurdles. As businesses seek to harness the potential of self-learning neuromorphic technologies, they grapple with the task of integrating these advanced systems into their operations efficiently. Addressing this challenge requires collaborative efforts between technology developers and businesses to establish standardized protocols and interfaces, simplifying the integration process. Additionally, investment in comprehensive training programs and educational initiatives is crucial to equipping professionals with the necessary skills to navigate the complexities of neuromorphic system integration effectively.
Scalability and Resource Constraints
Scalability remains a significant challenge in the Global Self-Learning Neuromorphic Market. While neuromorphic systems offer unparalleled efficiency in processing complex tasks, their scalability to handle large-scale applications is a persistent concern. As the volume of data processed by AI applications continues to increase, self-learning neuromorphic systems must scale proportionally to meet these demands. However, developing scalable neuromorphic hardware architectures and algorithms that maintain performance efficiency presents a formidable challenge. Resource constraints, both in terms of computational power and memory bandwidth, further exacerbate this issue. Ensuring that self-learning neuromorphic systems can seamlessly scale to accommodate the growing needs of industries such as healthcare, finance, and autonomous vehicles requires ongoing research and innovation. Overcoming these scalability challenges necessitates the development of energy-efficient, high-performance neuromorphic chips and intelligent algorithms capable of distributing and managing computational tasks effectively across large-scale neural networks.
Ethical and Privacy Concerns
The proliferation of self-learning neuromorphic technologies raises ethical and privacy concerns that pose significant challenges to the market. As these systems gain the ability to learn from vast datasets, questions surrounding data privacy, consent, and potential misuse of sensitive information come to the forefront. Issues related to algorithmic bias, where AI systems inadvertently perpetuate and amplify societal prejudices present in training data, also demand careful consideration. Ethical dilemmas arise concerning the use of self-learning neuromorphic systems in surveillance, decision-making processes, and other applications where human lives and fundamental rights are at stake. Striking a balance between technological advancement and ethical considerations requires the implementation of stringent regulations, industry standards, and transparent guidelines. Collaboration between policymakers, technology developers, and ethicists is essential to establish frameworks that safeguard individuals' privacy and ensure the responsible deployment of self-learning neuromorphic technologies in various contexts.
High Development Costs and Return on Investment
Developing advanced self-learning neuromorphic technologies entails substantial research, development, and manufacturing costs. The complexity of neuromorphic hardware, the need for specialized expertise, and the iterative nature of research and experimentation contribute to high development expenses. Additionally, businesses investing in the implementation of self-learning neuromorphic systems face challenges in demonstrating a tangible return on investment (ROI) within a reasonable timeframe. Predicting the exact business impact of these innovative technologies, especially in industries where traditional computing solutions are already established, proves challenging. Companies must justify the significant initial investment with concrete evidence of improved efficiency, reduced operational costs, or enhanced customer experiences. Moreover, the evolving nature of AI technologies demands continuous updates and adaptations, further adding to the long-term financial commitments. Overcoming this challenge requires comprehensive cost-benefit analyses, strategic planning, and a focus on long-term value. Collaboration between technology providers and businesses is vital to developing flexible pricing models and financial incentives that encourage widespread adoption while ensuring a sustainable ROI for enterprises investing in self-learning neuromorphic technologies.
Key Market Trends
Accelerated Adoption in Healthcare
One prominent trend shaping the Global Self-Learning Neuromorphic Market is the accelerated adoption of these technologies in the healthcare sector. Self-learning neuromorphic systems are being increasingly integrated into medical applications, ranging from disease diagnosis to personalized treatment plans. These systems can process vast amounts of patient data, including medical records, imaging scans, and genetic information, to identify patterns and provide valuable insights. In diagnostic imaging, for instance, neuromorphic algorithms enhance the accuracy of image interpretation, aiding clinicians in detecting anomalies and making more informed decisions. Moreover, neuromorphic computing plays a pivotal role in drug discovery by simulating biological processes and predicting the effectiveness of potential drug compounds. The healthcare industry’s rapid embrace of self-learning neuromorphic technologies not only improves patient outcomes but also drives market growth, with continuous innovation focused on addressing specific medical challenges.
Expansion in Autonomous Vehicles
The expansion of self-learning neuromorphic technologies in autonomous vehicles represents a significant market trend. These advanced systems are instrumental in enhancing the perception and decision-making capabilities of self-driving cars. Neuromorphic sensors and algorithms enable vehicles to interpret complex visual and sensory data in real-time, making split-second decisions critical for ensuring passenger safety. By mimicking human brain functions, these technologies improve object recognition, enabling vehicles to detect pedestrians, obstacles, and other vehicles accurately. Additionally, self-learning neuromorphic systems facilitate predictive analysis, allowing autonomous vehicles to anticipate and respond proactively to changing road conditions. As the automotive industry continues to invest in autonomous driving technologies, the integration of self-learning neuromorphic systems is poised to become standard, driving the market forward and reshaping the future of transportation.
Enhanced Human-Machine Interaction
A notable market trend is the focus on enhancing human-machine interaction through self-learning neuromorphic technologies. These systems enable natural language processing, gesture recognition, and emotional analysis, creating more intuitive and responsive human-computer interfaces. Virtual assistants and chatbots powered by neuromorphic algorithms can understand context and emotions, providing users with personalized and empathetic interactions. In addition, neuromorphic-based interfaces enhance the user experience in various applications, from smartphones and smart home devices to customer service platforms. The ability to interpret subtle cues and gestures enables a new level of communication between humans and machines, fostering deeper connections and more meaningful interactions. As businesses across industries prioritize customer engagement and user experience, the integration of self-learning neuromorphic technologies into interactive interfaces continues to gain momentum, driving market growth and innovation.
Growth in Edge Computing Applications
The Global Self-Learning Neuromorphic Market is witnessing a substantial trend towards the growth of edge computing applications. Edge computing refers to the processing of data closer to the source of data generation, reducing latency and enabling real-time decision-making. Self-learning neuromorphic technologies, with their ability to process information efficiently in real-time, are well-suited for edge computing environments. These systems are increasingly deployed in edge devices such as IoT sensors, cameras, and industrial equipment. By enabling localized, intelligent data processing, self-learning neuromorphic systems enhance the capabilities of edge devices, enabling them to operate autonomously and respond instantaneously to changing conditions. This trend is particularly relevant in applications where low latency and real-time decision-making are crucial, such as in smart cities, industrial automation, and healthcare monitoring. The integration of self-learning neuromorphic technologies into edge computing architectures optimizes data processing, improves operational efficiency, and drives market growth in these emerging sectors.
Rise of Neuromorphic Chips and Hardware Innovations
A key trend in the Global Self-Learning Neuromorphic Market is the rise of neuromorphic chips and hardware innovations. Advances in semiconductor technology have led to the development of specialized neuromorphic chips designed to efficiently process neural networks. These chips are optimized for the parallel processing requirements of self-learning algorithms, enabling faster and more energy-efficient computations. Moreover, there is a trend towards the integration of neuromorphic capabilities into traditional processors, creating hybrid architectures that combine the strengths of both approaches. Hardware innovations also include the development of neuromorphic sensors capable of capturing complex sensory data, such as touch and smell, further expanding the applications of self-learning neuromorphic technologies. These hardware advancements drive the market by offering more powerful and versatile solutions, encouraging the widespread adoption of self-learning neuromorphic systems across diverse industries. As technology developers continue to push the boundaries of hardware capabilities, the market is expected to experience a surge in innovative applications, paving the way for a new era of intelligent computing.
Segmental Insights
Vertical Insights
The Healthcare sector emerged as the dominant segment in the Global Self-Learning Neuromorphic Market. The Healthcare vertical experienced a substantial surge in the adoption of self-learning neuromorphic technologies due to their transformative impact on diagnostics, personalized treatment plans, and healthcare management. Neuromorphic systems proved instrumental in analyzing vast and complex medical datasets, enabling accurate disease diagnosis, drug discovery, and patient monitoring. The healthcare industry embraced these technologies for applications such as medical imaging interpretation, predictive analytics, and real-time patient data analysis, enhancing the efficiency of healthcare services. With the growing demand for AI-driven healthcare solutions, the Healthcare sector's dominance is expected to continue throughout the forecast period. The ongoing need for advanced technologies to improve patient outcomes, optimize healthcare workflows, and enhance overall healthcare delivery ensures the sustained prominence of self-learning neuromorphic applications in the Healthcare vertical. As healthcare providers and organizations prioritize data-driven decision-making and innovative medical solutions, the Healthcare segment is anticipated to maintain its dominance, driving the Global Self-Learning Neuromorphic Market in the coming years.
Application Insights
The Image Recognition segment emerged as the dominant force in the Global Self-Learning Neuromorphic Market. The surge in demand for advanced image recognition technologies across diverse industries, including healthcare, automotive, and surveillance, propelled this segment to the forefront. Self-learning neuromorphic systems, with their ability to mimic human visual processing, found extensive applications in tasks such as facial recognition, object detection, and image analysis. Businesses and organizations increasingly leveraged these systems to enhance security measures, improve diagnostic accuracy in healthcare, and optimize manufacturing processes. The robust adoption of self-learning neuromorphic technology for image recognition purposes not only addressed specific industry challenges but also showcased the potential for innovative applications, reinforcing the segment's dominance.
Moreover, the Image Recognition segment is poised to maintain its supremacy during the forecast period. As industries continue to invest in AI-driven solutions, the demand for precise and efficient image recognition capabilities is expected to grow. Neuromorphic systems, with their capacity for continuous learning and adaptation, are well-suited to handle the complexities of image recognition tasks, providing accurate results in real-time. This ongoing trend is fueled by the need for automation, data-driven decision-making, and enhanced customer experiences. As a result, businesses are anticipated to further integrate self-learning neuromorphic technologies into their image recognition applications, ensuring the sustained dominance of the Image Recognition segment in the Global Self-Learning Neuromorphic Market throughout the forecast period.
Regional Insights
North America emerged as the dominant region in the Global Self-Learning Neuromorphic Market. The region experienced significant advancements in artificial intelligence technologies, coupled with substantial investments in research and development. North American countries, particularly the United States and Canada, housed leading technology companies, research institutions, and innovative startups focusing on neuromorphic computing. These factors, along with a robust ecosystem supporting technological innovation, contributed to the region's dominance. Furthermore, the early adoption of self-learning neuromorphic technologies across various sectors, including healthcare, automotive, and defense, bolstered North America's market position. The presence of key market players, coupled with favorable government initiatives supporting AI research and development, further propelled the region's leadership. As the demand for AI-driven solutions continued to rise across industries, North America's well-established infrastructure, coupled with ongoing technological advancements, ensured its dominance in the Global Self-Learning Neuromorphic Market in 2022. The region is anticipated to maintain its leadership during the forecast period, driven by continuous investments in AI technologies, strong industry collaborations, and a conducive environment for innovation and market growth.
Recent Developments
- InSeptember 2023, Intel unveiled its groundbreaking series of self-learningneuromorphic processors, marking a significant advancement in the field ofartificial intelligence. These cutting-edge processors leverage neuromorphiccomputing principles, mimicking the human brain's synaptic connections toenable unparalleled learning and decision-making capabilities. Integrated withadvanced machine learning algorithms, Intel's neuromorphic processors excel inprocessing complex data patterns, making them ideal for applications inrobotics, autonomous vehicles, and real-time data analytics. The launchsignifies a crucial milestone in the Global Self-Learning Neuromorphic Market,demonstrating Intel's commitment to driving innovation in intelligent computingsolutions. With their ability to adapt and learn from diverse datasets, theseprocessors are poised to revolutionize industries, ushering in a new era ofintelligent automation and data-driven decision-making.
- InJanuary 2023, NVIDIA, a leading technology company, introduced its latestseries of self-learning neuromorphic GPUs, pushing the boundaries ofcomputational capabilities. These GPUs are engineered to handle intricateneural networks, enabling seamless integration with AI applications acrossvarious sectors. With enhanced parallel processing capabilities, NVIDIA'sneuromorphic GPUs deliver remarkable performance in deep learning tasks,including image recognition, natural language processing, and autonomousnavigation. The launch underscores the growing demand for high-performancecomputing solutions in the Global Self-Learning Neuromorphic Market, cateringto industries seeking faster and more efficient AI processing. NVIDIA'sinnovative approach not only accelerates AI research and development but alsopaves the way for transformative applications in healthcare, finance, andscientific research.
- InJune 2023, Qualcomm, a leading semiconductor and telecommunications equipmentcompany, unveiled its next-generation self-learning neuromorphic chips tailoredfor edge computing applications. These chips are designed to operateefficiently in resource-constrained environments, making them ideal for IoTdevices, smart sensors, and edge computing nodes. Leveraging neuromorphicprinciples, Qualcomm's chips enable intelligent data processing at the edge,reducing latency and enhancing real-time decision-making capabilities. Thelaunch addresses the growing trend of edge computing in the GlobalSelf-Learning Neuromorphic Market, meeting the demands of industries requiringrapid data analysis and localized AI inference. Qualcomm's focus onenergy-efficient, high-performance neuromorphic chips positions them as keyplayers in the emerging landscape of intelligent edge devices.
- InAugust 2023, IBM, a pioneer in cognitive computing, introduced itscomprehensive suite of self-learning neuromorphic software solutions, targetingdiverse industry applications. IBM's software offerings include advanced neuralnetwork libraries, development frameworks, and simulation tools, empoweringbusinesses to create customized self-learning applications. These solutionsfacilitate the development of intelligent chatbots, predictive maintenancesystems, and adaptive cybersecurity measures. IBM's entry into the softwaresegment of the Global Self-Learning Neuromorphic Market highlights the crucialrole of software development in maximizing the potential of neuromorphichardware. By providing robust tools and frameworks, IBM enables businesses toharness the power of self-learning algorithms, fostering innovation and drivingthe adoption of neuromorphic technologies across sectors.
Key Market Players
- IBMCorporation
- Intel Corporation
- Qualcomm Technologies, Inc.
- BrainChip Holdings Ltd.
- General Vision Inc.
- HRL Laboratories, LLC
- Hewlett Packard Enterprise DevelopmentLP
- Samsung Electronics Co., Ltd.
- Applied Brain Research Inc.
- Vicarious FPC Inc.
- Numenta Inc.
- Cerebras Systems Inc.
By Vertical | By Application | By Region |
|
|
|
Related Reports
- Sports Officiating Technologies Market Size - By Technology (Video-based, Sensor-based, Tracking, Communication), By App...
- Cognitive Computing Market Size - By Technology (Machine Learning, Natural Language Processing (NLP), Human Computer Int...
- Cognitive Computing Market Size - By Technology (Machine Learning, Natural Language Processing (NLP), Human Computer In...
- Decentralized Identity Market Size, By Identity Type (Biometrics, Non-Biometrics), By Enterprise Size (Large Enterprise,...
- Blockchain Identity Management Market Size - By Offering (Software, Service), By Provider Type (Application Provider, Mi...
- PayTV Market - By Technology (Cable TV, Satellite TV, Internet Protocol TV), By Subscription Type (Postpaid, Prepaid), ...
Table of Content
To get a detailed Table of content/ Table of Figures/ Methodology Please contact our sales person at ( chris@marketinsightsresearch.com )
List Tables Figures
To get a detailed Table of content/ Table of Figures/ Methodology Please contact our sales person at ( chris@marketinsightsresearch.com )
FAQ'S
For a single, multi and corporate client license, the report will be available in PDF format. Sample report would be given you in excel format. For more questions please contact:
Within 24 to 48 hrs.
You can contact Sales team (sales@marketinsightsresearch.com) and they will direct you on email
You can order a report by selecting payment methods, which is bank wire or online payment through any Debit/Credit card, Razor pay or PayPal.
Discounts are available.
Hard Copy