Artificial Intelligence in Diabetes Management Market - Global Industry Size, Share, Trends, Opportunity, and Forecast, 2018-2028 Segmented By Device (Diagnostic Devices, Glucose Monitoring Devices, Insulin Delivery Devices), By Technique (Case-Based Reasoning, Intelligent Data Analysis), By Region, and By Competition
Published Date: November - 2024 | Publisher: MIR | No of Pages: 320 | Industry: Healthcare | Format: Report available in PDF / Excel Format
View Details Buy Now 2890 Download Sample Ask for Discount Request CustomizationForecast Period | 2024-2028 |
Market Size (2022) | USD 550.41 million |
CAGR (2023-2028) | 12.15% |
Fastest Growing Segment | Glucose Monitoring Devices |
Largest Market | North America |
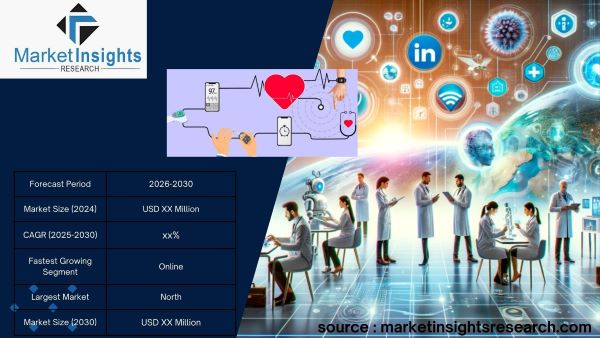
Market Overview
Global Artificial Intelligence in Diabetes Management Market has valued at USD 550.41 million in 2022 and is anticipated to project impressive growth in the forecast period with a CAGR of 12.15% through 2028. The Global Artificial Intelligence in Diabetes Management Market refers to the use of artificial intelligence (AI) technologies in the management and treatment of diabetes. AI has been increasingly employed in healthcare to enhance the accuracy and efficiency of diagnosis, monitoring, and treatment of various diseases, including diabetes.
Key Market Drivers
Rising Diabetes Prevalence
Diabetes, often referred to as a global epidemic, has been steadily on the rise for several decades. According to the International Diabetes Federation (IDF), an estimated 463 million adults were living with diabetes in 2019, a number projected to increase to 700 million by 2045. This alarming increase in diabetes prevalence presents a significant challenge to healthcare systems worldwide. However, it also presents a unique opportunity for the development and adoption of Artificial Intelligence (AI) in diabetes management.
With the growing number of individuals at risk of developing diabetes, early diagnosis and risk prediction have become critical. AI-powered algorithms can analyze vast datasets, including medical records and genetic information, to identify individuals at high risk of diabetes. This proactive approach allows healthcare providers to intervene early, potentially preventing or delaying the onset of the disease. As a result, the demand for AI-driven diagnostic tools and risk assessment models is on the rise.
Managing diabetes is not a one-size-fits-all approach. Each individual's response to treatment varies, making personalized treatment plans essential. AI algorithms can analyze a patient's unique health data, including glucose levels, medication history, and lifestyle factors, to create personalized treatment plans. These plans optimize medication regimens, dietary recommendations, and exercise routines, leading to better glycemic control. As diabetes prevalence increases, the demand for tailored, AI-driven treatment plans is set to grow.
Continuous Glucose Monitoring (CGM) devices, integrated with AI algorithms, are revolutionizing diabetes management. These devices provide real-time data on blood glucose levels, allowing individuals with diabetes and their healthcare providers to make informed decisions about insulin dosages, diet adjustments, and exercise routines. As more people seek efficient and accurate ways to manage their diabetes, the demand for CGM solutions powered by AI is expected to surge.
The rise of telemedicine and remote monitoring solutions is closely tied to the increasing prevalence of diabetes. AI-enhanced telemedicine platforms enable healthcare providers to remotely monitor patients with diabetes, reducing the need for frequent in-person visits. This not only improves patient convenience but also ensures timely interventions and support. As the diabetic population continues to grow, so does the demand for convenient and accessible care, driving the adoption of AI in telemedicine.
Managing diabetes involves monitoring various aspects of a patient's health, including glucose levels, blood pressure, and medication adherence. AI excels at integrating data from diverse sources, providing healthcare professionals with a comprehensive view of a patient's health. This integrated approach enables better decision-making and coordination of care, addressing the complexity of diabetes management in an increasingly prevalent population.
Continuous Glucose Monitoring (CGM)
Diabetes management is an ongoing challenge for millions of people worldwide. Continuous Glucose Monitoring (CGM) has emerged as a game-changer in diabetes care, providing real-time data on blood glucose levels. When combined with Artificial Intelligence (AI), CGM technology offers unparalleled opportunities to enhance diabetes management.
Traditionally, individuals with diabetes relied on periodic fingerstick tests to monitor blood glucose levels. CGM systems, on the other hand, provide a continuous stream of glucose data throughout the day and night. This real-time data is invaluable for both patients and healthcare providers, allowing for timely adjustments to insulin dosages, diet, and exercise. As the demand for accurate and up-to-the-minute information on glucose levels grows, CGM technology becomes a key driver for AI adoption in diabetes management.
CGM combined with AI has the potential to revolutionize glycemic control. AI algorithms can analyze CGM data to identify patterns and trends in glucose levels. These patterns can inform treatment decisions, helping individuals with diabetes achieve tighter control over their blood sugar. Improved glycemic control not only reduces the risk of complications but also enhances the quality of life for those with diabetes.
AI-powered CGM systems can provide personalized insights into diabetes management. By analyzing CGM data along with other health and lifestyle information, AI can create tailored treatment plans. These plans consider individual factors such as medication history, meal preferences, and activity levels. The ability to provide highly customized guidance is a significant driver for the adoption of AI in diabetes management.
Beyond managing diabetes, AI-enhanced CGM can play a preventive role. Machine learning algorithms can identify early signs of glycemic fluctuations that may lead to complications. This proactive approach enables healthcare providers to intervene before serious problems develop, potentially preventing hospitalizations and improving patient outcomes. As the focus on preventive care grows, AI-powered CGM technology becomes increasingly important.
CGM, coupled with AI, supports telemedicine and remote monitoring in diabetes management. Patients can share their CGM data with healthcare providers in real-time, facilitating remote consultations and adjustments to treatment plans. This not only reduces the burden on healthcare systems but also offers convenience to patients, particularly in rural or underserved areas.
AI excels at integrating data from various sources. CGM systems generate a wealth of data, including glucose levels, meal timing, and physical activity. AI can consolidate this data with electronic health records, medication history, and lifestyle factors, providing a comprehensive view of a patient's health. Such data integration streamlines decision-making and enhances the overall diabetes management process.
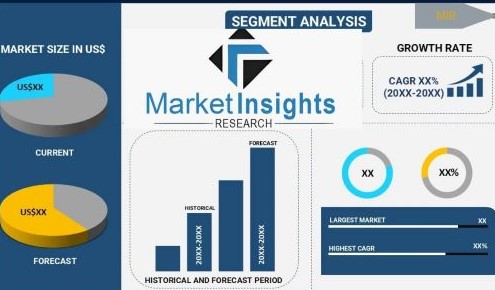
Predictive Analytics
The prevalence of diabetes is on the rise worldwide, creating an urgent need for more effective and efficient ways to manage this chronic condition. Predictive Analytics, when combined with Artificial Intelligence (AI), is emerging as a powerful tool in the field of diabetes management.
Predictive Analytics utilizes AI algorithms to analyze extensive datasets, including patient health records, genetic information, and lifestyle factors. By identifying patterns and correlations, these algorithms can predict an individual's risk of developing diabetes or prediabetes. Early detection and risk assessment are crucial in combating the rising prevalence of diabetes, as they enable healthcare providers to intervene proactively and provide personalized preventive measures.
One of the critical challenges in diabetes management is tailoring treatment plans to individual patients. Predictive Analytics enhances the personalization of these plans by taking into account an individual's specific health metrics, medication history, dietary preferences, and activity levels. This precision in treatment recommendations improves patient compliance and ultimately contributes to better glycemic control.
Diabetes is associated with various complications, including neuropathy, retinopathy, and cardiovascular diseases. Predictive Analytics can analyze patient data to predict the likelihood of these complications developing. By identifying high-risk patients, healthcare providers can implement preventive measures, offer specialized care, and closely monitor those at risk, potentially reducing the incidence and severity of complications.
Managing diabetes often involves adjusting medication regimens. Predictive Analytics can analyze a patient's glucose trends and medication response over time. This data-driven approach enables healthcare providers to optimize medication dosages and types for each patient, reducing the risk of hypoglycemia and hyperglycemia episodes.
The rise of telehealth and remote monitoring is transforming diabetes care, and Predictive Analytics plays a pivotal role. These systems continuously collect patient data, including glucose levels, activity, and vital signs. AI-driven predictive models can analyze this real-time data to detect deviations from the norm, prompting timely interventions by healthcare providers. Remote monitoring offers convenience for patients and can help reduce the strain on healthcare systems.
On a broader scale, Predictive Analytics can be used to identify trends and patterns in diabetes prevalence within specific populations. Public health organizations and policymakers can leverage this information to allocate resources, design targeted interventions, and implement preventive strategies. This population-level approach can contribute to reducing the overall burden of diabetes.
In the realm of diabetes research, Predictive Analytics is invaluable. It can analyze vast datasets from clinical trials to identify potential biomarkers, treatment responses, and patient subgroups. This information accelerates the development of new therapies and interventions for diabetes management.
Data Integration
The prevalence of diabetes continues to escalate worldwide, making it one of the most pressing healthcare challenges of our time. Fortunately, technological advancements are offering new solutions, and one of the key enablers of progress is data integration. When combined with Artificial Intelligence (AI), data integration plays a pivotal role in transforming diabetes management.
Data integration in diabetes management involves the aggregation and analysis of diverse data sources, including electronic health records (EHRs), continuous glucose monitoring (CGM) systems, wearables, and patient-generated data. This integration provides healthcare providers with a comprehensive view of a patient's health, including glucose levels, medication history, lifestyle factors, and comorbidities. These holistic insights enable more informed decision-making and a more accurate understanding of the patient's overall health.
Individuals with diabetes have unique needs, and a one-size-fits-all approach to treatment often falls short. Data integration allows AI algorithms to create personalized treatment plans based on a patient's specific health data. These plans consider factors such as glucose trends, medication responses, dietary habits, and activity levels. Personalization enhances the effectiveness of treatment, improving glycemic control and reducing the risk of complications.
The real-time nature of data integration is invaluable in diabetes management. AI algorithms continuously analyze patient data, detecting anomalies and patterns that may indicate impending issues such as hypoglycemia or hyperglycemia. Healthcare providers can receive alerts and intervene promptly, ensuring timely adjustments to insulin dosages or other treatment regimens. This proactive approach minimizes the risk of severe glycemic episodes.
Data integration supports remote monitoring and telehealth initiatives. Patients can share their data seamlessly with healthcare providers, facilitating remote consultations and reducing the need for in-person visits. Telehealth and remote monitoring improve patient engagement and compliance with treatment plans, particularly in underserved or rural areas.
In the realm of diabetes research, data integration accelerates progress. Researchers can access integrated datasets from clinical trials, real-world patient data, and genetic information to uncover new insights. AI algorithms analyze this data to identify potential biomarkers, treatment responses, and patient subgroups. Such information is invaluable in drug development and the discovery of innovative therapies.
At the population level, data integration enables public health organizations and policymakers to monitor diabetes trends and allocate resources effectively. By analyzing integrated data, authorities can identify high-risk populations, implement targeted interventions, and design preventive strategies. This proactive approach contributes to reducing the overall burden of diabetes.
Data integration promotes interoperability among healthcare systems and devices. This interoperability streamlines the flow of information, reducing duplication of efforts and improving the efficiency of healthcare delivery. Healthcare providers can access relevant patient data more easily, resulting in faster decision-making and improved patient care.
Key Market Challenges
Data Privacy and Security Concerns
One of the foremost challenges in implementing AI in diabetes management is the sensitive nature of patient data. AI algorithms rely on vast amounts of patient information, including medical records, genetic data, and lifestyle information. Ensuring the privacy and security of this data is paramount, and complying with stringent data protection regulations, such as the Health Insurance Portability and Accountability Act (HIPAA) in the United States or the General Data Protection Regulation (GDPR) in Europe, can be complex and costly.
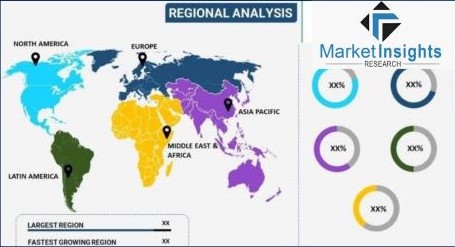
Data Quality and Standardization
The effectiveness of AI algorithms depends on the quality and consistency of the data they analyze. Variations in data formats, completeness, and accuracy can hinder the performance of AI systems. Achieving data standardization and ensuring data quality across different healthcare systems and devices remain significant challenges.
Interoperability
Interoperability challenges persist in the healthcare sector, with various devices, systems, and software often unable to communicate seamlessly. For AI to reach its full potential in diabetes management, it must integrate with various healthcare systems, electronic health records, and wearable devices. Achieving this interoperability remains an ongoing challenge.
Key Market Trends
AI-Powered Predictive Analytics
Predictive analytics will play a central role in the future of diabetes management. AI algorithms will leverage vast datasets, including patient records, genomic data, and lifestyle information, to predict the risk of diabetes, forecast glycemic fluctuations, and identify potential complications. This proactive approach will empower healthcare providers to intervene early, preventing adverse events and improving patient outcomes.
Enhanced Personalization
Personalization will continue to be a driving force in diabetes care. AI will analyze individual patient data to create highly personalized treatment plans, encompassing medication management, dietary recommendations, and exercise regimens. As AI becomes more sophisticated, these plans will adapt in real-time to accommodate changes in the patient's health and lifestyle.
Continuous Glucose Monitoring (CGM) Advancements
The CGM market will witness significant advancements with the integration of AI. CGM devices, combined with AI algorithms, will offer real-time analysis of glucose data, helping individuals make immediate decisions about their treatment and lifestyle choices. AI will also improve the accuracy and reliability of CGM systems, making them an indispensable tool in diabetes management.
Segmental Insights
Device Insights
Based on the category of Device, Glucose monitoring devices are poised to capture a substantial market share in the Global Artificial Intelligence in Diabetes Management Market during the forecast period for several compelling reasons. Firstly, the rising prevalence of diabetes worldwide has spurred the demand for advanced tools to manage the condition effectively. Secondly, the integration of artificial intelligence (AI) into glucose monitoring devices has enhanced their accuracy and efficiency, enabling real-time data analysis and personalized treatment recommendations. Moreover, the growing adoption of wearable and IoT-based healthcare technologies has made continuous glucose monitoring more accessible and convenient for patients. As a result, these devices are anticipated to play a pivotal role in the evolution of diabetes management, making them a prominent player in the market's growth trajectory.
Technique Insights
Intelligent data analysis is poised to claim a substantial market share in the Global Artificial Intelligence in Diabetes Management Market during the forecast period for several compelling reasons. Firstly, the sheer volume of data generated by diabetes patients, including glucose levels, lifestyle data, and medical history, necessitates advanced analytical tools to extract meaningful insights. Secondly, the application of artificial intelligence in data analysis enables healthcare professionals to identify patterns, predict glucose fluctuations, and customize treatment plans more effectively, ultimately improving patient outcomes. Furthermore, the continuous advancements in AI algorithms and machine learning techniques are making data analysis more accurate and efficient, driving the adoption of intelligent data analysis solutions in diabetes management. As the demand for precision medicine and personalized care grows, intelligent data analysis is expected to play a pivotal role in shaping the future of diabetes management, solidifying its prominence in the market.
Regional Insights
North America is poised to dominate the Global Artificial Intelligence in Diabetes Management Market for several compelling reasons. Firstly, the region boasts a robust healthcare infrastructure and high healthcare expenditure, fostering the adoption of cutting-edge technologies such as AI in diabetes management. Secondly, North America has a significant prevalence of diabetes, and the region is proactive in addressing this health challenge. This has led to substantial investments in research and development, with many companies and research institutions pioneering innovative AI solutions for diabetes care. Additionally, a favorable regulatory environment and a strong focus on patient-centric healthcare have accelerated the integration of AI into diabetes management practices in North America. The presence of well-established AI and technology companies in the region also contributes to its leadership in this market. All these factors combined position North America at the forefront of the Global Artificial Intelligence in Diabetes Management Market.
Key Market Players
- Vodafone Group PLC
- Apple Inc
- GoogleInc
- InternationalBusiness Machines Corporation (IBM)
- GlookoInc
- TidepoolInc
By Device | By Technique | By Region |
|
|
|
Related Reports
- Veterinary Infusion Pumps Market – By Product (Syringe, Volumetric, Ambulatory, Implantable), Animal type (Companion, ...
- Veterinary CRO and CDMO Market – By Animal Type (Companion, Livestock), Service Type (CRO, [Clinical Trials, Quality A...
- Pet Sitting Services Market – By Service (In-home Pet Sitting Services, Drop-in Visits, Boarding Services, Daycare Ser...
- Veterinary Video Endoscopes Market – By Product (Equipment, PACS Software), Animal Type (Small, Large), Application (D...
- Pet Herbal Supplements Market – By Product Type (Multivitamins & Minerals, Omega 3 Fatty Acids), Application (Digestiv...
- Equine Artificial Insemination Market Size - By Component (Services, Semen [Fresh, Chilled, Frozen], Equipment, Reagents...
Table of Content
To get a detailed Table of content/ Table of Figures/ Methodology Please contact our sales person at ( chris@marketinsightsresearch.com )
List Tables Figures
To get a detailed Table of content/ Table of Figures/ Methodology Please contact our sales person at ( chris@marketinsightsresearch.com )
FAQ'S
For a single, multi and corporate client license, the report will be available in PDF format. Sample report would be given you in excel format. For more questions please contact:
Within 24 to 48 hrs.
You can contact Sales team (sales@marketinsightsresearch.com) and they will direct you on email
You can order a report by selecting payment methods, which is bank wire or online payment through any Debit/Credit card, Razor pay or PayPal.
Discounts are available.
Hard Copy